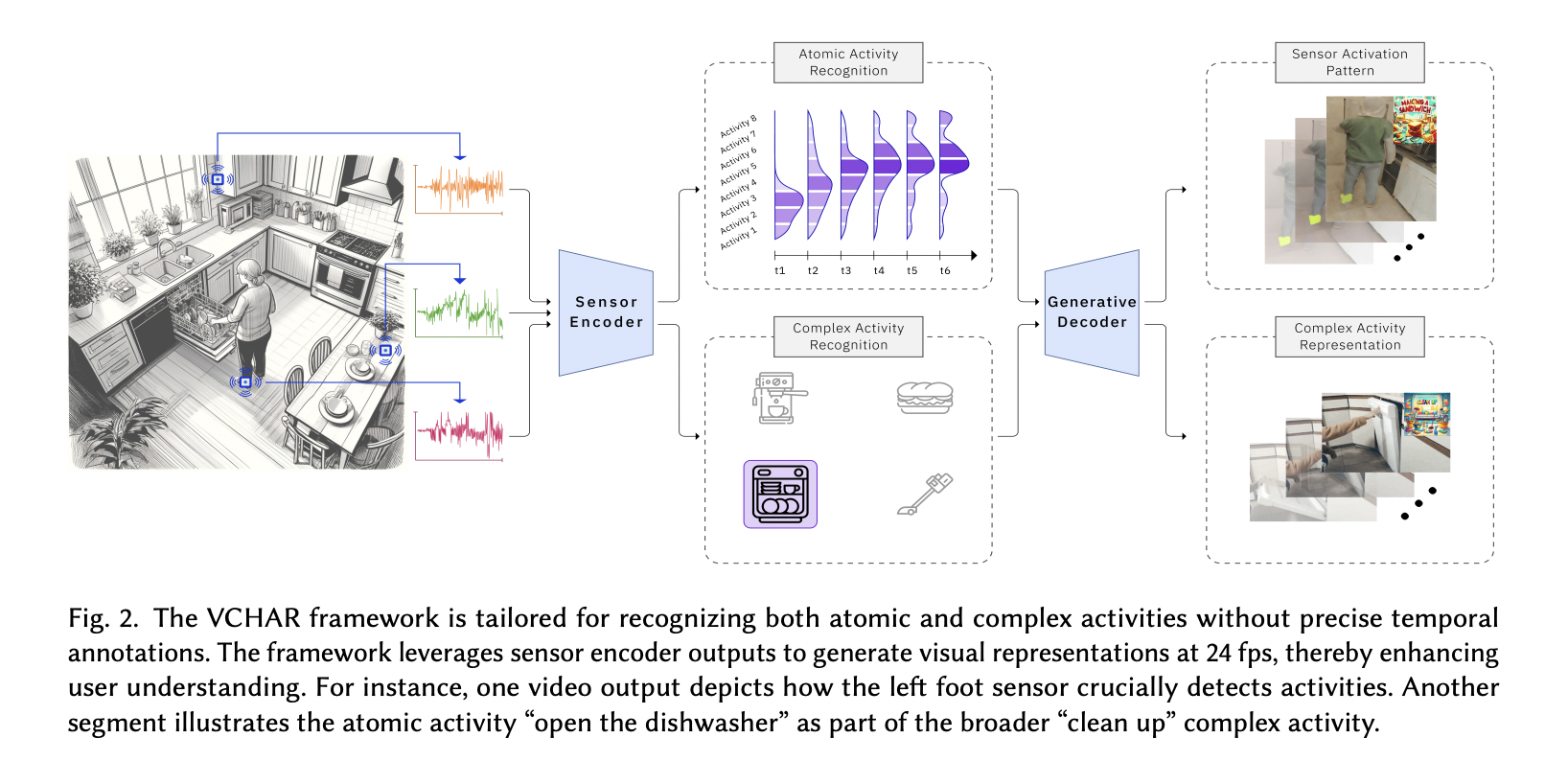
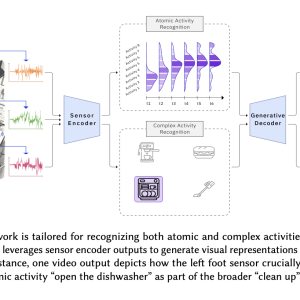
Complex Human Activity Recognition (CHAR) in ubiquitous computing, particularly in smart environments, presents significant challenges due to the labor-intensive and error-prone process of labeling datasets with precise temporal information of atomic activities. This task becomes impractical in real-world scenarios where accurate and detailed labeling is scarce. The need for effective CHAR methods that do not rely on meticulous labeling is crucial for advancing applications in healthcare, elderly care, surveillance, and emergency response.
Traditional CHAR methods typically require detailed labeling of atomic activities within specific time intervals to train models effectively. These methods often involve segmenting data to improve accuracy, which is labor-intensive and prone to inaccuracies. In practice, many datasets only indicate the types of activities occurring within specific collection intervals without precise temporal or sequential labeling, leading to combinatorial complexity and potential errors in labeling.
To address these issues, a team of researchers from Rutgers University propose the Variance-Driven Complex Human Activity Recognition (VCHAR) framework. VCHAR leverages a generative approach to treat atomic activity outputs as distributions over specified intervals, thus eliminating the need for precise labeling. This framework utilizes generative methodologies to provide intelligible explanations for complex activity classifications through video-based outputs, making it accessible to users without prior machine learning expertise.
The VCHAR framework employs a variance-driven approach that utilizes the Kullback-Leibler divergence to approximate the distribution of atomic activity outputs within specific time intervals. This method allows for the recognition of decisive atomic activities without the need to eliminate transient states or irrelevant data. By doing so, VCHAR enhances the detection rates of complex activities even when detailed labeling of atomic activities is absent.
Furthermore, VCHAR introduces a novel generative decoder framework that transforms sensor-based model outputs into integrated visual domain representations. This includes visualizations of complex and atomic activities along with relevant sensor information. The framework uses a Language Model (LM) agent to organize diverse data sources and a Vision-Language Model (VLM) to generate comprehensive visual outputs. The authors also propose a pretrained “sensor-based foundation model” and a “one-shot tuning strategy” with masked guidance to facilitate rapid adaptation to specific scenarios. Experimental results on three publicly available datasets show that VCHAR performs competitively with traditional methods while significantly enhancing the interpretability and usability of CHAR systems.
The integration of a Language Model (LM) and a Vision-Language Model (VLM) allows for the synthesis of comprehensive, coherent visual narratives that represent the detected activities and sensor information. This capability not only aids in better understanding and trust in the system’s outputs but also enhances the ability to communicate findings to stakeholders who may not have a technical background.
The VCHAR framework effectively addresses the challenges of CHAR by eliminating the need for precise labeling and providing intelligible visual representations of complex activities. This innovative approach improves the accuracy of activity recognition and makes the insights accessible to non-experts, bridging the gap between raw sensor data and actionable information. The framework’s adaptability, achieved through pre-training and one-shot tuning, makes it a promising solution for real-world smart environment applications that require accurate and contextually relevant activity recognition and description.
Check out the Paper. All credit for this research goes to the researchers of this project. Also, don’t forget to follow us on Twitter. Join our Telegram Channel and LinkedIn Group.
If you like our work, you will love our newsletter.. Don’t Forget to join our 46k+ ML SubReddit
If You are interested in a promotional partnership (content/ad/newsletter), please fill out this form.
The post VCHAR: A Novel Artificial Intelligence AI Framework that Treats the Outputs of Atomic Activities as a Distribution Over Specified Intervals appeared first on MarkTechPost.