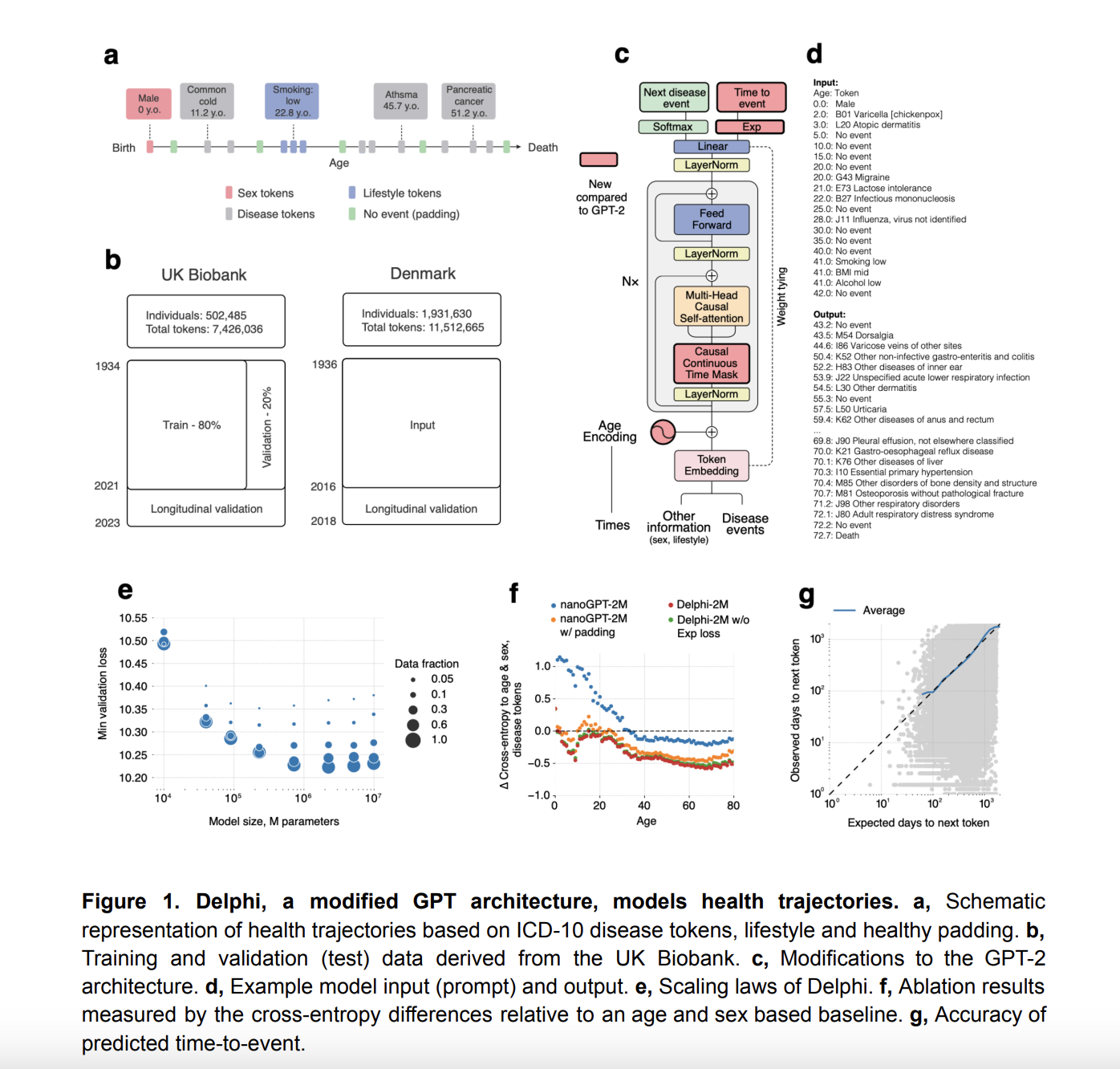
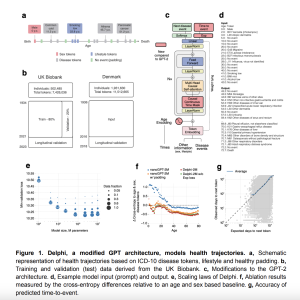
AI holds significant potential to revolutionize healthcare by predicting disease progression using vast health records, thus enabling personalized care. Understanding multi-morbidity—clusters of chronic and acute conditions influenced by lifestyle, genetics, and socioeconomic factors—is crucial for tailored healthcare and preventive measures. Despite existing prediction algorithms for specific diseases, there is a gap in comprehensive models that can predict a broad range of conditions. Recent advancements, such as transformer models inspired by LLMs, promise to overcome these challenges by modeling complex temporal dependencies in health data. However, the full potential of these models in multi-morbidity prediction remains largely unexplored.
Researchers from various institutions have developed Delphi-2M, an advanced AI model based on the GPT architecture, to predict disease progression in large populations. Based on data from 400,000 UK Biobank participants, Delphi-2M predicts over 1,000 diseases and deaths by analyzing past health records, demographics, and lifestyle factors. It generates detailed future health trajectories for individuals and provides insights into disease clusters and their time-dependent impacts. Validated against 1.9 million Danish records without parameter changes, Delphi-2M accurately models population health and reveals how past events shape future health outcomes, making it a robust tool for personalized healthcare prediction.
Delphi-2M, an AI model, accurately predicts the incidence of over 1,000 diseases, aligning closely with observed age and sex trends. It effectively models varied disease patterns in a validation cohort, such as childhood chickenpox peaks and age-related rises in other conditions. Delphi-2M’s predictions, continuously updated with new data, show significant inter-individual variability for diseases like septicemia. With AUCs averaging 0.8, its performance rivals established risk models like Framingham for cardiovascular disease. Delphi-2M’s calibration and longitudinal validation with UK Biobank data confirm its reliability in forecasting short-term and long-term disease trajectories, offering comprehensive multi-disease predictions.
Generative models like Delphi-2M can predict future disease trajectories based on past medical histories. Evaluating 100,000 sampled trajectories from the UK Biobank, Delphi-2M accurately mirrored observed disease rates and incidences up to age 70. With an average accuracy of 17% in the first year, decreasing to 14% over 20 years, Delphi-2M surpasses basic age-sex models. It distinguishes high- and low-risk groups, effectively predicting disease burdens over two decades. Moreover, Delphi-2M’s generated synthetic trajectories, which do not duplicate training data, have practical uses, such as training new models, thereby preserving data privacy and broadening potential applications.
Delphi, a modified GPT-2 model, is designed to predict health trajectories by analyzing sequences of top-level ICD-10 diagnoses supplemented with lifestyle data like sex, BMI, smoking, and alcohol use. Training data from the UK Biobank and external validation using Danish health records were employed. Delphi replaces GPT-2’s discrete positional encoding with a continuous age-based encoding and introduces an additional head to predict the time between events. This allows Delphi to accurately model the timing and sequence of health events, surpassing standard GPT models in predicting disease onset and progression.
Delphi-2M, a GPT-2-based model, predicts the progression of multiple diseases by learning patterns from health data of over 1,000 diseases in 400,000 UK Biobank participants. It excels in predicting disease trajectories and estimating cumulative disease burdens over long periods. Tested on Danish health data, it proved adaptable without further training. While effective, it inherits biases from its training data and must be used cautiously. Delphi-2M’s flexible architecture allows for future integration of additional health data like genomics and wearables, making it a promising tool for healthcare planning, personalized medicine, and understanding complex disease interactions.
Check out the Paper and Code. All credit for this research goes to the researchers of this project. Also, don’t forget to follow us on Twitter.
Join our Telegram Channel and LinkedIn Group.
If you like our work, you will love our newsletter..
Don’t Forget to join our 45k+ ML SubReddit
The post Delphi-2M: A Modified GPT Architecture for Modeling Future Health Based on Past Medical History appeared first on MarkTechPost.