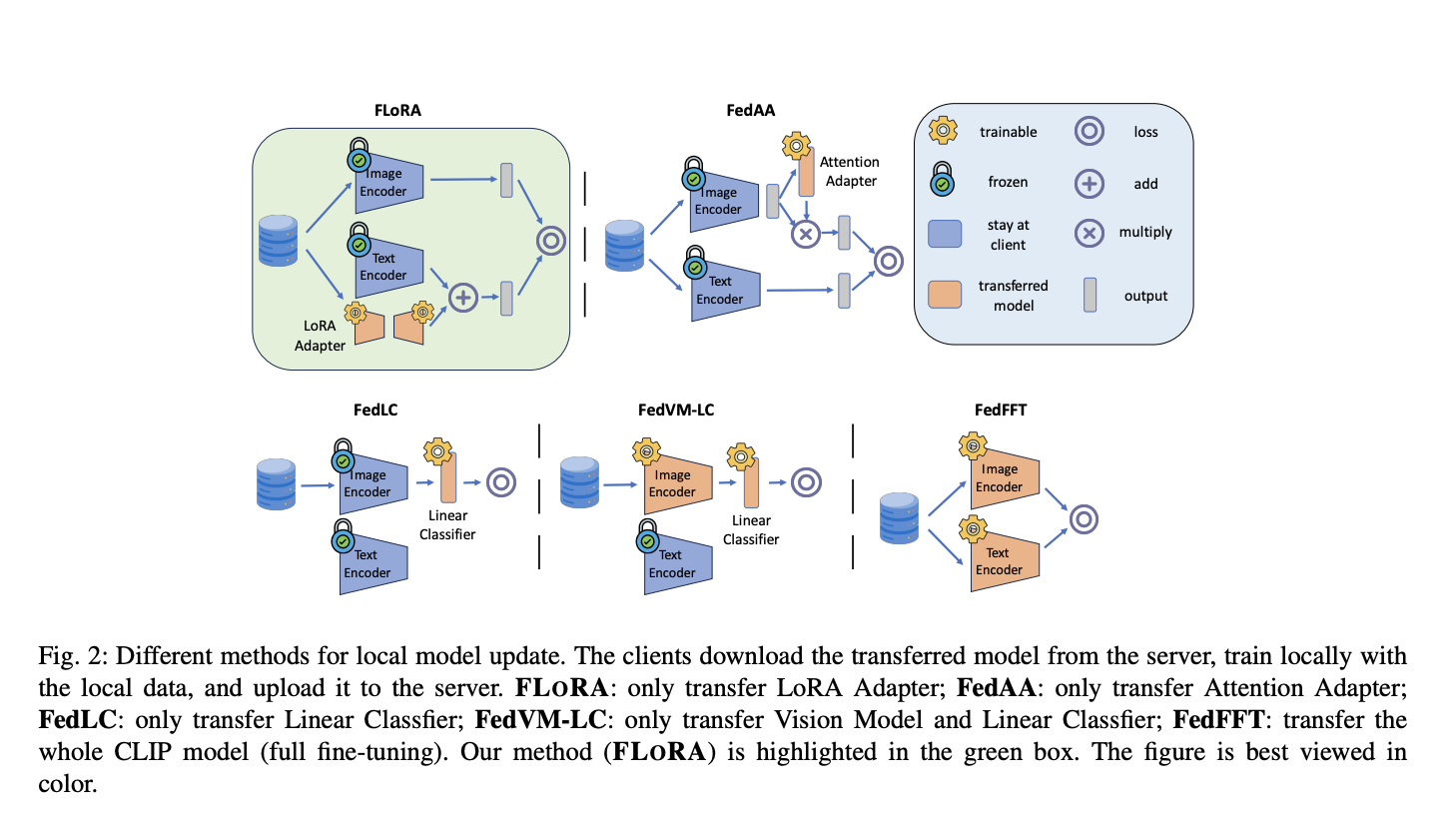
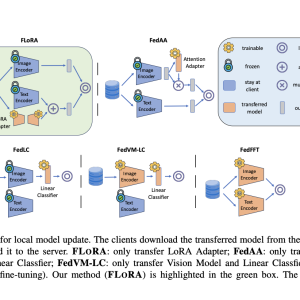
Traditional methods for training vision-language models (VLMs) often require the centralized aggregation of vast datasets, which raises concerns regarding privacy and scalability. Federated learning offers a solution by allowing models to be trained across a distributed network of devices while keeping data locally but adapting VLMs to this framework presents unique challenges.
To address these challenges, a team of researchers from Intel Corporation and Iowa State University introduced FLORA (Federated Learning with Low-Rank Adaptation) to address the challenge of training vision-language models (VLMs) in federated learning (FL) settings while preserving data privacy and minimizing communication overhead. FLORA fine-tunes VLMs like the CLIP model by utilizing parameter-efficient adapters, namely Low-Rank Adaptation (LoRA), in conjunction with Federated Learning. Instead of requiring centralized data mining, FLORA enables model training across decentralized data sources while preserving data privacy and minimizing communication costs. By selectively updating only a small subset of the model’s parameters using LoRA, FLORA accelerates training time and reduces memory usage compared to full fine-tuning.
The FLORA method uses LoRA-adapted CLIP models for client-side training and local updates. An Adam optimizer helps with gradient-based optimization. A server then aggregates these updates using a weighted averaging technique similar to FedAvg. The Low-Rank Adaptation (LoRA) method is a key part of FLORA’s success because it adds trainable low-rank matrices to certain layers of a model that has already been trained. This cuts down on the amount of work that needs to be done and the amount of memory that is needed. FLORA improves performance and adapts models more efficiently in federated learning settings by adding LoRA to the CLIP model.
Experimental evaluations demonstrate FLORA’s effectiveness across various datasets and learning environments. FLORA consistently outperforms traditional FL methods in both IID and non-IID settings, demonstrating superior accuracy and adaptability. Also, FLORA’s efficiency analysis shows that it uses much less memory and communication compared to baseline methods, which shows that it could be used in real-world federated learning situations. A few-shot evaluation further confirms FLORA’s proficiency in managing data scarcity and distribution variability, showcasing its robust performance even with limited training examples.
In conclusion, FLORA presents a promising solution to the challenge of training vision-language models in federated learning settings. By leveraging Federated Learning and Low-Rank Adaptation, FLORA enables efficient model adaptation while preserving data privacy and minimizing communication overhead. The methodology’s performance across various datasets and learning environments underscores its potential to revolutionize federated learning for VLMs. The superior accuracy, efficiency, and adaptability that FLORA can achieve makes it a strong solution for dealing with the difficulties of real-world data challenges in distributed learning environments.
Check out the Paper. All credit for this research goes to the researchers of this project. Also, don’t forget to follow us on Twitter. Join our Telegram Channel, Discord Channel, and LinkedIn Group.
If you like our work, you will love our newsletter..
Don’t Forget to join our 40k+ ML SubReddit
The post This AI Paper Proposes FLORA: A Novel Machine Learning Approach that Leverages Federated Learning and Parameter-Efficient Adapters to Train Visual-Language Models VLMs appeared first on MarkTechPost.