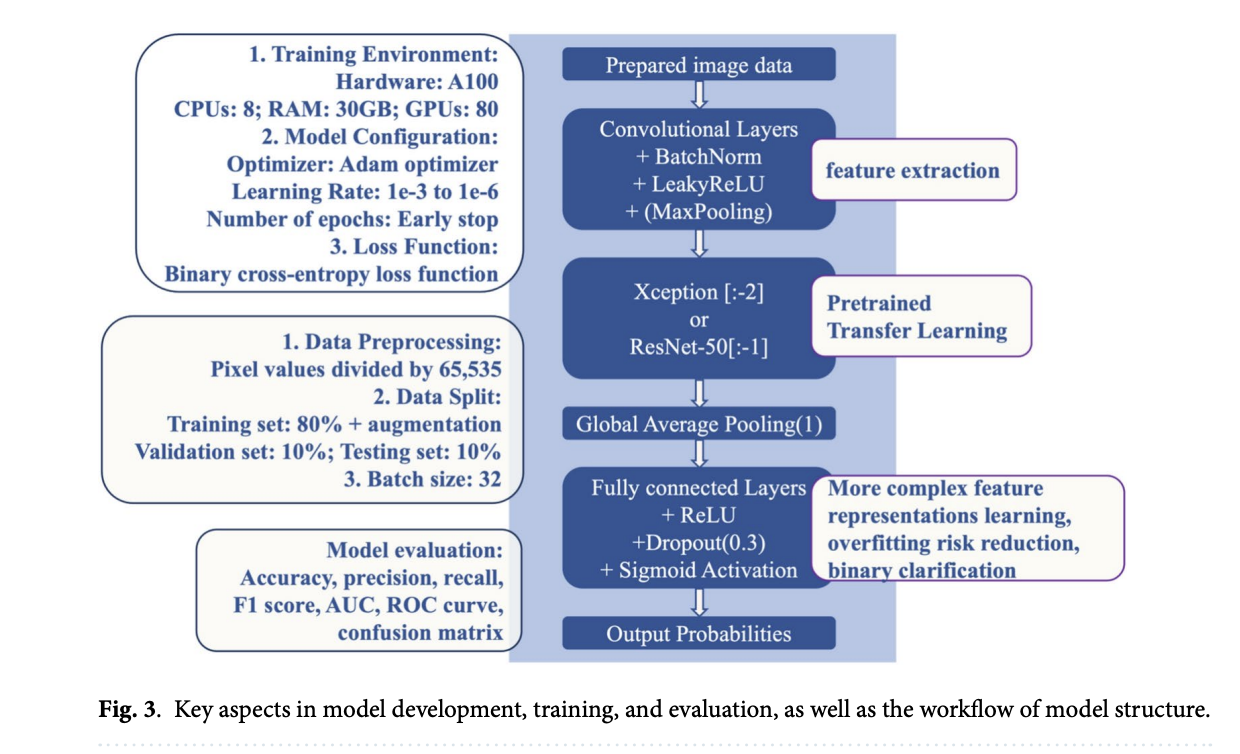
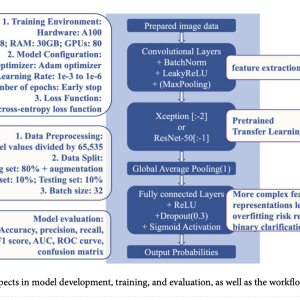
Accessible mammography datasets and advanced machine-learning methods are key to enhancing computer-aided breast cancer diagnosis. However, limited access to private datasets, selective image sampling from public databases, and partial code availability hinder these models’ reproducibility and validation. These limitations create barriers for researchers aiming to advance in this field. Breast cancer causing 670,000 deaths worldwide in 2022. Although technologies like tomosynthesis improve screening, false positives and variability in radiologists’ interpretations raise patient anxiety and healthcare costs. Additionally, CAD algorithms face challenges in reliability due to limited datasets and reduced performance in real-world applications.
Researchers from Biomedical Deep Learning LLC and Washington University in St. Louis have developed a pilot codebase to streamline the entire process of breast cancer diagnosis, from image preprocessing to model development and evaluation. The team identified that larger input sizes enhance malignancy detection accuracy across various model types using the CBIS-DDSM mass subset, which provides full images and regions of interest (ROIs). This codebase is designed to advance global breast cancer diagnostic software development efforts by providing a reproducible framework incorporating recent innovations.
The CBIS-DDSM dataset contains publicly accessible mammography images curated by trained experts, with segmentation and pathology labeling updates. The images were converted from DICOM to PNG format and processed to maintain the abnormal region’s central focus, including applying image transformations for augmentation. The model training pipeline includes data loading, normalization, and a tailored convolutional neural network architecture, followed by validation using accuracy, precision, recall, F1 score, and AUROC metrics. Performance tracking through early stopping and checkpointing ensures optimized results, facilitating future research and improvements in diagnostic accuracy.
The study explored the CBIS-DDSM mass subset dataset to improve breast cancer diagnostics through image processing and deep learning. The subset includes 1,696 abnormal ROIs and 1,592 corresponding full mammograms in DICOM format, which were converted to PNG for analysis. Each image was processed to focus on abnormal regions, standardized to 598×598 pixels, and enhanced through data augmentation techniques. The augmented images were split for training (80%), validation (10%), and testing (10%), with models built using transfer learning and evaluated on multiple image sizes—224×224, 299×299, 448×448, and 598×598 pixels. The study highlighted that using larger image sizes improved the detection of malignant cases, underscoring the importance of preserving image detail in medical imaging.
Model performance varied based on architecture and input size, with ResNet-50 models outperforming Xception models, particularly at 448×448 pixels, where the former achieved a higher ROC AUC score and malignant detection rate. Larger images enabled more detailed representations, beneficial for capturing specific cancerous features, while smaller pictures led to some detail loss, affecting detection rates. The study concluded that ResNet-50’s architecture, which captures intricate patterns through residual learning, performed effectively for mammography tasks compared to Xception’s depthwise convolution approach, making it a stronger choice for detecting fine-grained malignancies in mammography images.
In conclusion, Breast cancer screening models have evolved through diverse innovations, from simulating cancer progression to applying AI techniques like CAD and federated learning. However, inconsistent methodologies and opaque datasets create challenges in replicability. To address this, the study contributes a fully accessible codebase—from image preprocessing to evaluation—using the CBIS-DDSM dataset. This codebase provides a transparent workflow to support model development and validation in breast cancer diagnosis. By enhancing input size and applying stringent quality controls, the researchers aim to improve model accuracy and reliability, encouraging transparency and accelerating advancements in the field.
Check out the Paper. All credit for this research goes to the researchers of this project. Also, don’t forget to follow us on Twitter and join our Telegram Channel and LinkedIn Group. If you like our work, you will love our newsletter.. Don’t Forget to join our 55k+ ML SubReddit.
[AI Magazine/Report] Read Our Latest Report on ‘SMALL LANGUAGE MODELS‘
The post Enhancing Breast Cancer Diagnosis: A Transparent, Reproducible Workflow Using CBIS-DDSM and Advanced Machine Learning Techniques appeared first on MarkTechPost.