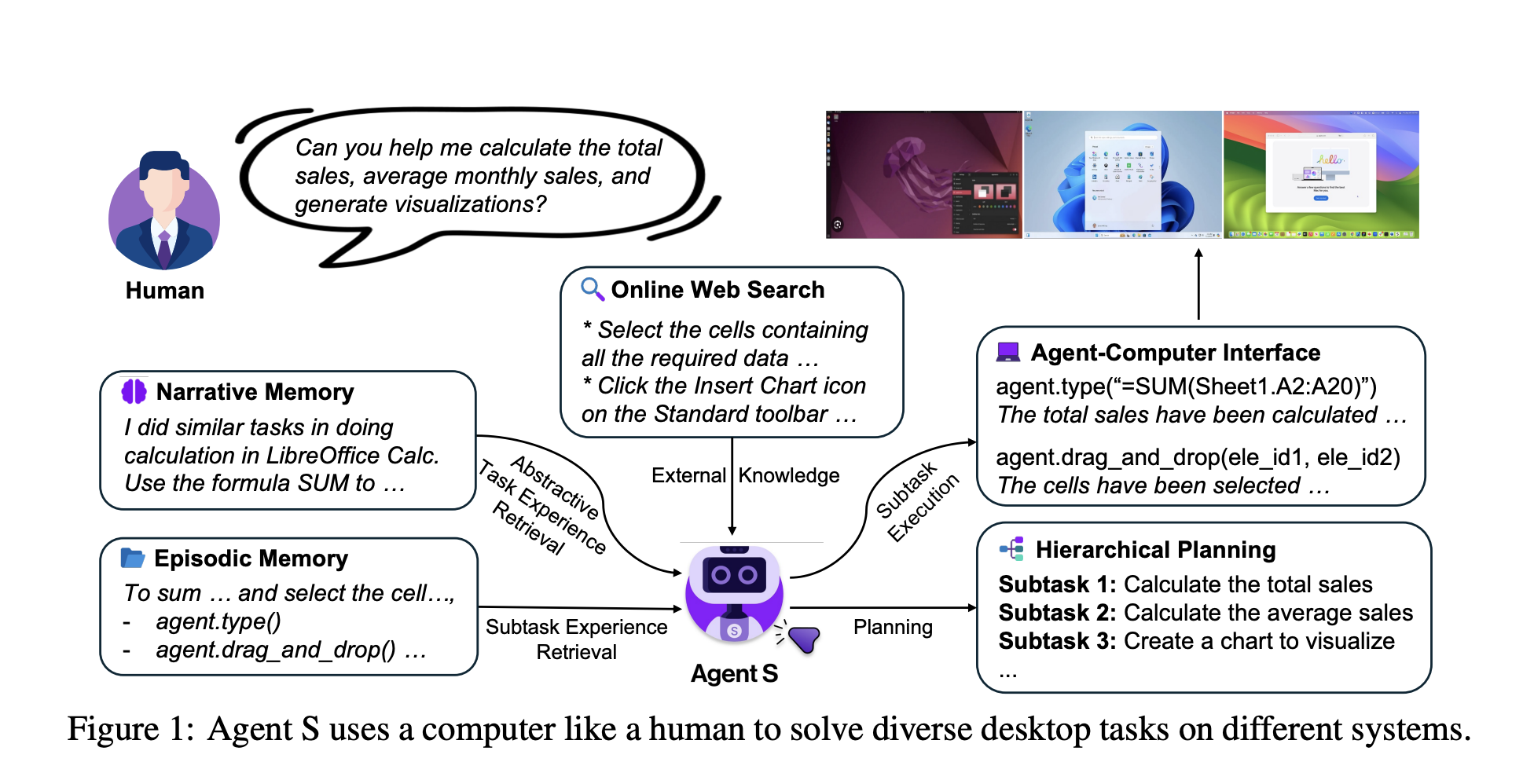
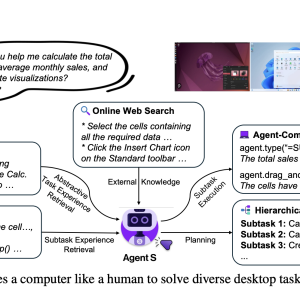
The challenge lies in automating computer tasks by replicating human-like interaction, which involves understanding varied user interfaces, adapting to new applications, and managing complex sequences of actions similar to how a human would perform them. Current solutions struggle with handling complex and varied interfaces, acquiring and updating domain-specific knowledge, and planning multi-step tasks that require precise sequences of actions. Additionally, agents must learn from diverse experiences, adapt to new environments, and effectively handle dynamic and inconsistent user interfaces.
Simular Research introduces Agent S, an open agentic framework designed to use computers like a human, specifically through autonomous interaction with GUIs. This framework aims to transform human-computer interaction by enabling AI agents to use the mouse and keyboard as humans would to complete complex tasks. Unlike conventional methods that require specialized scripts or APIs, Agent S focuses on interaction with the GUI itself, providing flexibility across different systems and applications. The core novelty of Agent S lies in its use of experience-augmented hierarchical planning, allowing it to learn from both internal memory and online external knowledge to decompose large tasks into subtasks. An advanced Agent-Computer Interface (ACI) facilitates efficient interactions by using multimodal inputs.
The structure of Agent S is composed of several interconnected modules working in unison. At the heart of Agent S is the Manager module, which combines information from online searches and past task experiences to devise comprehensive plans for completing a given task. This hierarchical planning strategy allows the breakdown of a large, complex task into smaller, manageable subtasks. To execute these plans, the Worker module uses episodic memory to retrieve relevant experiences for each subtask. A self-evaluator component is also employed, summarizing successful task completions into narrative and episodic memories, allowing Agent S to continuously learn and adapt. The integration of an advanced ACI further facilitates interactions by providing the agent with a dual-input mechanism: visual information for understanding context and an accessibility tree for grounding its actions to specific GUI elements.
The results presented in the paper highlight the effectiveness of Agent S across various tasks and benchmarks. Evaluations on the OSWorld benchmark showed a significant improvement in task completion rates, with Agent S achieving a success rate of 20.58%, representing a relative improvement of 83.6% compared to the baseline. Additionally, Agent S was tested on the WindowsAgentArena benchmark, demonstrating its generalizability across different operating systems without explicit retraining. Ablation studies revealed the importance of each component in enhancing the agent’s capabilities, with experience augmentation and hierarchical planning being critical to achieving the observed performance gains. Specifically, Agent S was most effective in tasks involving daily or professional use cases, outperforming existing solutions due to its ability to retrieve relevant knowledge and plan efficiently.
In conclusion, Agent S provides a significant advancement in the development of autonomous GUI agents by integrating hierarchical planning, an Agent-Computer Interface, and a memory-based learning mechanism. This framework demonstrates that by using a combination of multimodal inputs and leveraging past experiences, AI agents can effectively use computers like humans to accomplish a variety of tasks. The approach not only simplifies the automation of multi-step tasks but also broadens the scope of AI agents by improving their adaptability and task generalization capabilities across different environments. Future work aims to address the number of steps and time efficiency of the agent’s actions to enhance its practicality in real-world applications further.
Check out the Paper and GitHub. All credit for this research goes to the researchers of this project. Also, don’t forget to follow us on Twitter and join our Telegram Channel and LinkedIn Group. If you like our work, you will love our newsletter.. Don’t Forget to join our 50k+ ML SubReddit.
[Upcoming Live Webinar- Oct 29, 2024] The Best Platform for Serving Fine-Tuned Models: Predibase Inference Engine (Promoted)
The post Simular Research Introduces Agent S: An Open-Source AI Framework Designed to Interact Autonomously with Computers through a Graphical User Interface appeared first on MarkTechPost.