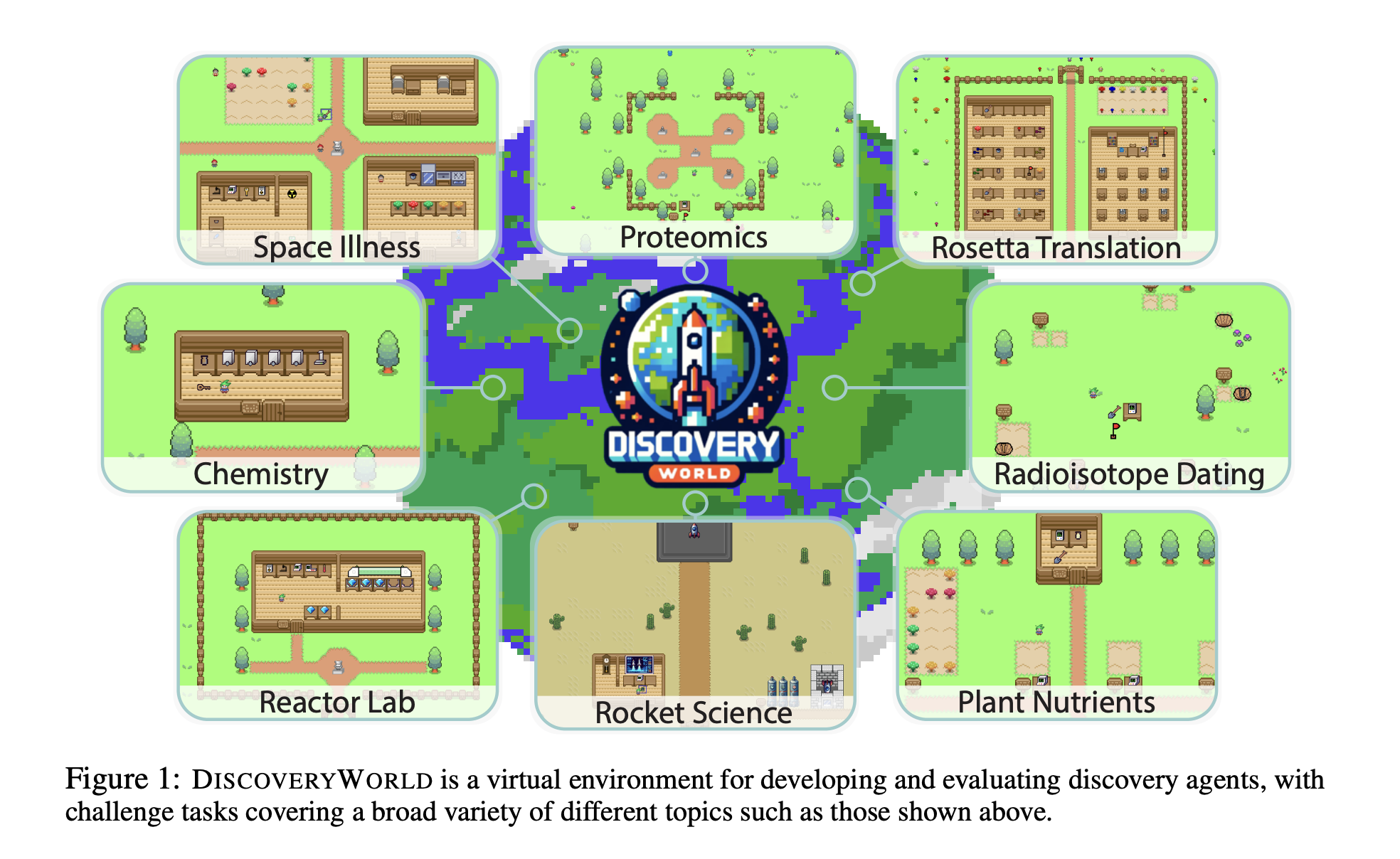
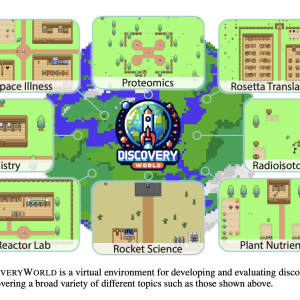
Automated scientific discovery has the potential to enhance progress across various scientific fields significantly. However, assessing an AI agent’s ability to use comprehensive scientific reasoning is challenging due to the high costs and impracticalities of conducting real-world experiments. While recent neural techniques have led to successful discovery systems for specific problems like protein folding, mathematics, and materials science, these systems typically circumvent the complete discovery process, focusing instead on systematic searches within a predefined hypothesis space. This raises the question of how much more can be accomplished if AI is utilized throughout the scientific process, including creativity, hypothesis generation, and experimental design.
Recent advancements in real-world discovery systems have shown promise in fields like genetics, chemistry, and proteomics, though these systems are often costly and tailored to specific tasks. Various virtual environments have been developed for robotics, exploration, and scientific discovery, including AI2-Thor and NetHack. However, many of these environments prioritize entertainment over rigorous scientific exploration. While some, like ScienceWorld, tackle basic science challenges, they typically need more comprehensive processes for thorough scientific discovery. Overall, existing systems vary in complexity and application, often emphasizing narrow task performance rather than fostering broad scientific research skills.
Researchers from the Allen Institute, Microsoft Research, and the University of Arizona have developed DISCOVERYWORLD, a pioneering virtual environment designed for agents to conduct full cycles of scientific discovery. This text-based platform features 120 challenges across eight diverse topics, such as rocket science and proteomics, emphasizing developing general discovery skills rather than task-specific solutions. The environment allows agents to hypothesize, experiment, analyze, and draw conclusions. Additionally, a robust evaluation framework measures agent performance through task completion and relevant actions, revealing that existing agents face significant challenges in this new context, highlighting the environment’s potential to advance AI discovery capabilities.
The DISCOVERYWORLD simulator uses a custom engine to create dynamic discovery simulations with varied object properties and behaviors. It consists of approximately 20,000 lines of Python code using the Pygame framework, featuring an API for agent development and a graphical interface for human interaction. The environment is organized as a 32 × 32 tile grid, where agents receive observations in text and visual formats. The simulation includes 14 possible actions and parametrically generated tasks across eight discovery themes with three difficulty levels. Evaluation metrics assess task completion, process adherence, and the accuracy of discovered knowledge, enabling a comprehensive performance evaluation of agents.
The study analyzes the performance of strong baseline agents and human scientists on DISCOVERYWORLD tasks, focusing on zero-shot generalization for iterative scientific discovery. The study evaluates three baseline models in a zero-shot setting across 120 tasks, each assessed independently. Performance metrics include task completion and explanatory knowledge discovery. Results indicate that while baseline agents show varying levels of success, there is a notable performance gap compared to human scientists. Eleven human participants with relevant scientific backgrounds were recruited to provide insights into human performance.
The performance of human participants in discovery tasks varied widely, with completion rates ranging from tasks solved by all to those tackled by only one participant. On average, humans achieved a 66% completion rate, while their knowledge performance was slightly lower at 55%, sometimes resorting to brute-force solutions without providing explanatory insights. In contrast, baseline agent performance was subpar, with the best agent, REACT, completing only 38% of easy tasks and 18% of challenge tasks. DISCOVERYWORLD is a pioneering virtual platform for assessing agents’ capabilities in scientific discovery, highlighting the need for improved general AI discovery agents.
Check out the Paper. All credit for this research goes to the researchers of this project. Also, don’t forget to follow us on Twitter and join our Telegram Channel and LinkedIn Group. If you like our work, you will love our newsletter.. Don’t Forget to join our 50k+ ML SubReddit
[Upcoming Event- Oct 17 202] RetrieveX – The GenAI Data Retrieval Conference (Promoted)
The post Meet DiscoveryWorld: A Virtual Environment for Developing and Benchmarking An Agent’s Ability to Perform Complete Cycles of Novel Scientific Discovery appeared first on MarkTechPost.