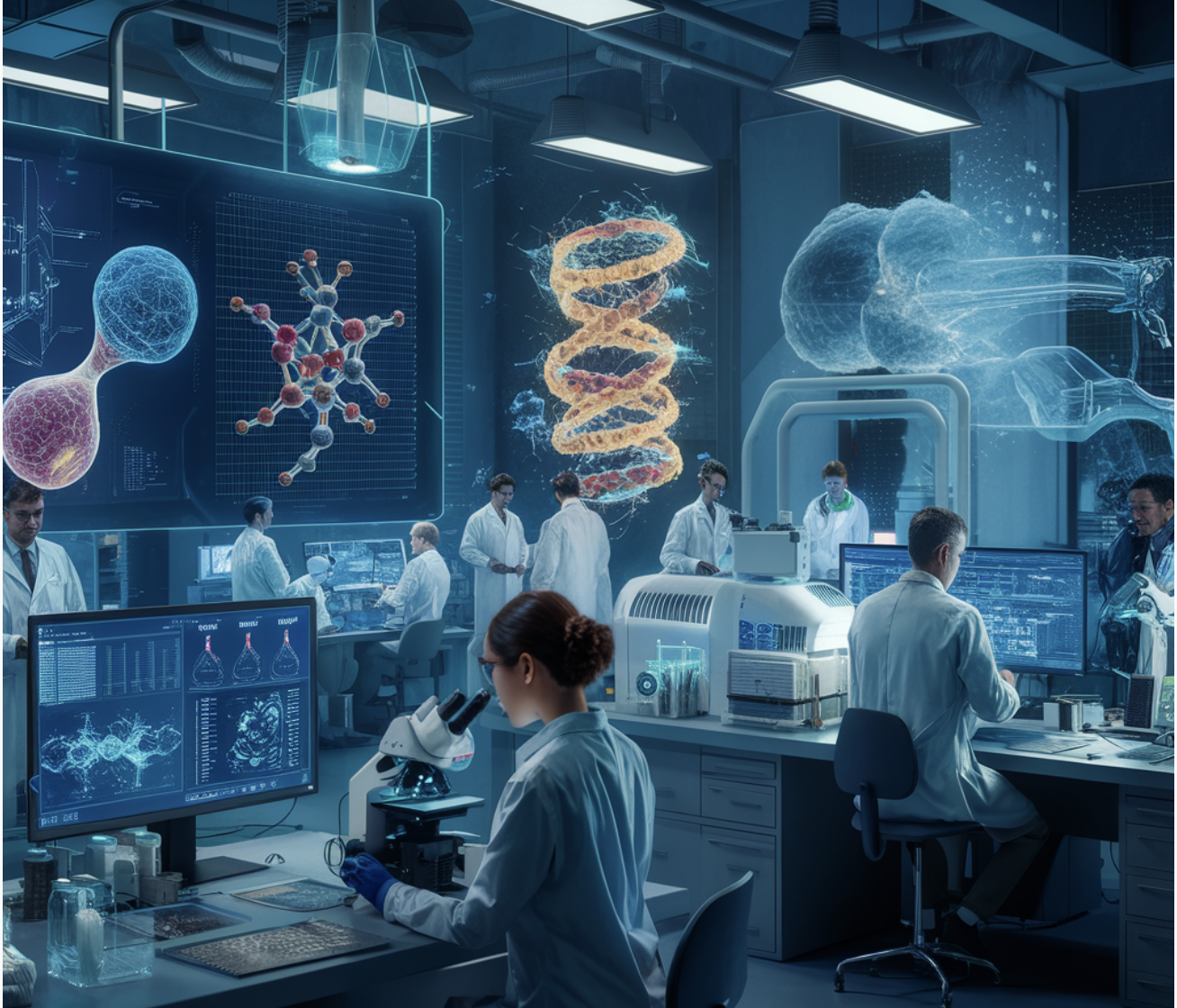
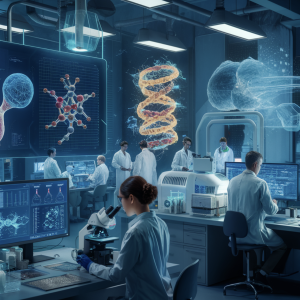
In recent years, the integration of ML and AI into biomedicine has become increasingly pivotal, particularly in digital health. The explosion of high-throughput technologies, such as genome-wide sequencing, extensive libraries of medical images, and large-scale drug perturbation screens, has resulted in vast and complex biomedical data. This multi-omics data offers a wealth of information that can be utilized to gain profound insights into the mechanisms of health and disease. By applying advanced ML techniques, including deep neural networks, to these data sets, researchers can perform tasks like automated disease classification, digital image recognition, and virtual drug screening with unprecedented accuracy. These advancements enhance our understanding of disease signatures and healthy baselines and pave the way for innovative treatments and personalized healthcare approaches.
The collaboration between AI and systems biology is transforming the advancement of precision medicine. This methodology customizes medical interventions for each patient by considering their genetic composition, environmental influences, and lifestyle factors. AI-driven methods are proving particularly transformative in infectious diseases and other complex conditions where traditional single-gene or protein biomarkers are insufficient. AI can provide precise diagnostics, optimize treatment strategies, and predict disease progression by processing and interpreting large, diverse datasets. This multidisciplinary approach fosters collaboration among experts from various fields, such as genomics, proteomics, and clinical data, ensuring that AI models are robust, reliable, and ethically sound. As the healthcare industry continues to embrace these technologies, ongoing research and collaboration will be crucial to overcoming challenges related to data privacy, regulatory compliance, and the integration of AI into clinical practice, ultimately leading to more personalized and proactive healthcare.
Synergies Between AI and Digital Pathology:
Advances in DL and image processing are revolutionizing digital pathology. Techniques like deep convolutional neural networks excel in classifying and diagnosing digitized whole-slide images. For instance, a network trained on over 100,000 skin disease images demonstrated diagnostic accuracy comparable to current standards. This technology could extend to mobile platforms for early cancer detection. In clinical settings, AI can analyze electronic health records to predict medical events and integrate multi-omics data to tailor treatment strategies, significantly enhancing precision oncology and personalized medicine.
AI and Single-Cell Analysis in Cancer Research:
ML and DL are pivotal in analyzing the complexity of cancer at the single-cell level. These technologies help decipher the diverse cellular environments within tumors and identify subtle genetic variations among cell populations. Techniques like single-cell RNA sequencing (scRNA-Seq) reveal cellular heterogeneity and are enhanced by clustering algorithms such as Louvain community detection. Spatial transcriptomics merges traditional histology with gene expression data, allowing precise mapping of disease pathology. ML models predict disease progression and treatment responses, providing insights into tumor dynamics and resistance mechanisms.
AI’s Role in Chemical Informatics and Drug Discovery:
AI transforms chemical informatics and drug discovery by enabling rapid prediction and analysis of drug targets and their biological activities. AI techniques can assess drug properties, such as absorption, distribution, metabolism, and toxicity (ADME-Tox), and facilitate the virtual screening of billions of molecules, streamlining the drug development process. Researchers can swiftly identify and synthesize potential drug candidates for biological testing by integrating vast chemical databases with AI and lab automation. Moreover, computational models can predict drug mechanisms, repurpose existing drugs, and optimize drug designs, significantly accelerating the journey from discovery to clinical application.
Integrative AI-Driven Strategies for Precision Medicine in Infectious Diseases:
The study explores the transformative impact of AI in precision medicine, particularly for infectious diseases. By integrating AI with comprehensive patient data—encompassing demographics, genetic profiles, and immune responses—personalized treatment plans are developed to optimize patient outcomes. The study involves a diverse cohort and compares AI-driven treatment against standard care. Advanced AI algorithms analyze multi-dimensional data, offering tailored therapeutic recommendations and enhancing drug discovery processes. The results highlight significant improvements in treatment efficacy and patient outcomes, underscoring AI’s potential to revolutionize personalized healthcare and disease management.
Conclusion:
The research underscores the transformative impact of AI in precision medicine, particularly for infectious diseases. AI’s integration enables personalized treatment by analyzing diverse patient data, such as age and genetic profiles, to tailor therapies that enhance efficacy and minimize side effects. Beyond patient care, AI streamlines the drug discovery process, identifying promising therapeutic candidates with unparalleled efficiency. AI’s applications extend to diagnostics, molecular pathology, and medical education, highlighting its broad influence. However, ethical considerations, data privacy, and technical challenges remain crucial issues to address as AI revolutionizes healthcare.
The post Advancements in AI: Transforming Precision Medicine Across Biomedicine appeared first on MarkTechPost.