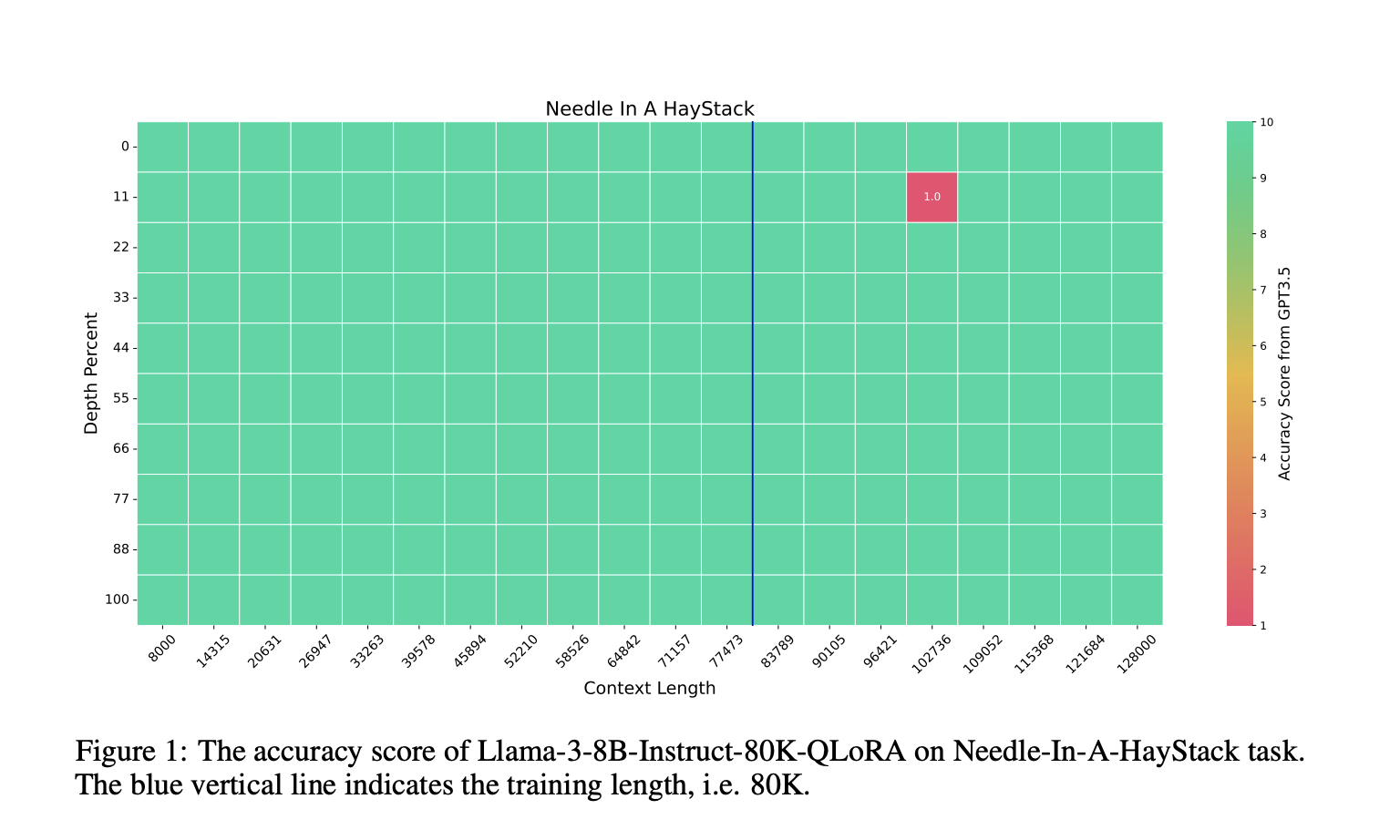
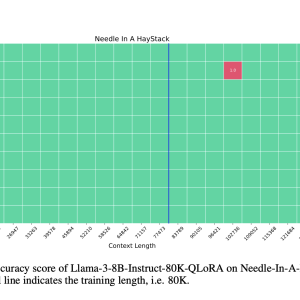
Natural language processing (NLP) focuses on enabling computers to understand and generate human language, making interactions more intuitive and efficient. Recent developments in this field have significantly impacted machine translation, chatbots, and automated text analysis. The need for machines to comprehend large amounts of text and provide accurate responses has led to the development of advanced language models that continuously push the boundaries of machine understanding.
Despite significant advancements in NLP, models often need to help maintain context over extended text and conversations, especially when the context includes lengthy documents. This leads to challenges in generating accurate and relevant responses. Moreover, these models are computationally expensive, making it difficult to deploy them in resource-constrained environments. There is a pressing need for models that are efficient and capable of understanding and maintaining context over long text sequences.
Existing research includes models like GPT, which excels at text generation and sentiment analysis, and BERT, known for its bidirectional training that improves context comprehension. T5 standardizes NLP tasks as text-to-text, while RoBERTa enhances BERT’s training process for superior performance. Despite their advancements, challenges persist regarding computational efficiency and context preservation in lengthy conversations, driving ongoing research to improve these models for more accurate and efficient language understanding.
Researchers from the Beijing Academy of Artificial Intelligence and the Renmin University of China have introduced Llama-3-8B-Instruct-80K-QLoRA, which significantly extends the context length of the original Llama-3 from 8K to 80K tokens. This proposed method stands out for preserving contextual understanding over long text sequences while reducing computational demands. Its unique approach leverages enhanced attention mechanisms and innovative training strategies, allowing it to handle longer contexts more efficiently than previous models.
The methodology uses GPT-4 to generate 3.5K training samples for Single-Detail QA, Multi-Detail QA, and Biography Summarization tasks. Researchers fine-tuned Llama-3-8B-Instruct-80K-QLoRA using QLoRA, which applies LoRA on projection layers while training the embedding layer. They incorporated RedPajama, LongAlpaca, and synthetic data to prevent forgetting and enhance contextual understanding. The training, completed on 8xA800 GPUs in 8 hours, involved organizing question-answer pairs into multi-turn conversations and then fine-tuning the entire dataset to improve long-context capabilities.
The model achieved a 100% accuracy rate in the Needle-In-A-Haystack task across its entire context length. In LongBench benchmarks, it consistently surpassed other models except in the code completion task. In InfBench tasks, it achieved 30.92% accuracy in the LongBookQA task, significantly outperforming other models while also performing well in summarization tasks. On the MMLU benchmark, it demonstrated strong performance, achieving competitive results in zero-shot evaluations and highlighting its superior ability to handle long-context tasks efficiently.
To conclude, the research introduced Llama-3-8B-Instruct-80K-QLoRA, a model that extends the context length of Llama-3 from 8K to 80K tokens. It addresses the challenge of maintaining context in long conversations by enhancing comprehension while reducing computational demands. The model’s performance across benchmarks like LongBench and InfBench demonstrated its ability to handle extensive text sequences accurately. This work advances NLP research by offering a model that efficiently understands and processes longer contexts, paving the way for more advanced language understanding applications.
Check out the Paper and GitHub. All credit for this research goes to the researchers of this project. Also, don’t forget to follow us on Twitter. Join our Telegram Channel, Discord Channel, and LinkedIn Group.
If you like our work, you will love our newsletter..
Don’t Forget to join our 40k+ ML SubReddit
The post This AI Paper Introduces Llama-3-8B-Instruct-80K-QLoRA: New Horizons in AI Contextual Understanding appeared first on MarkTechPost.