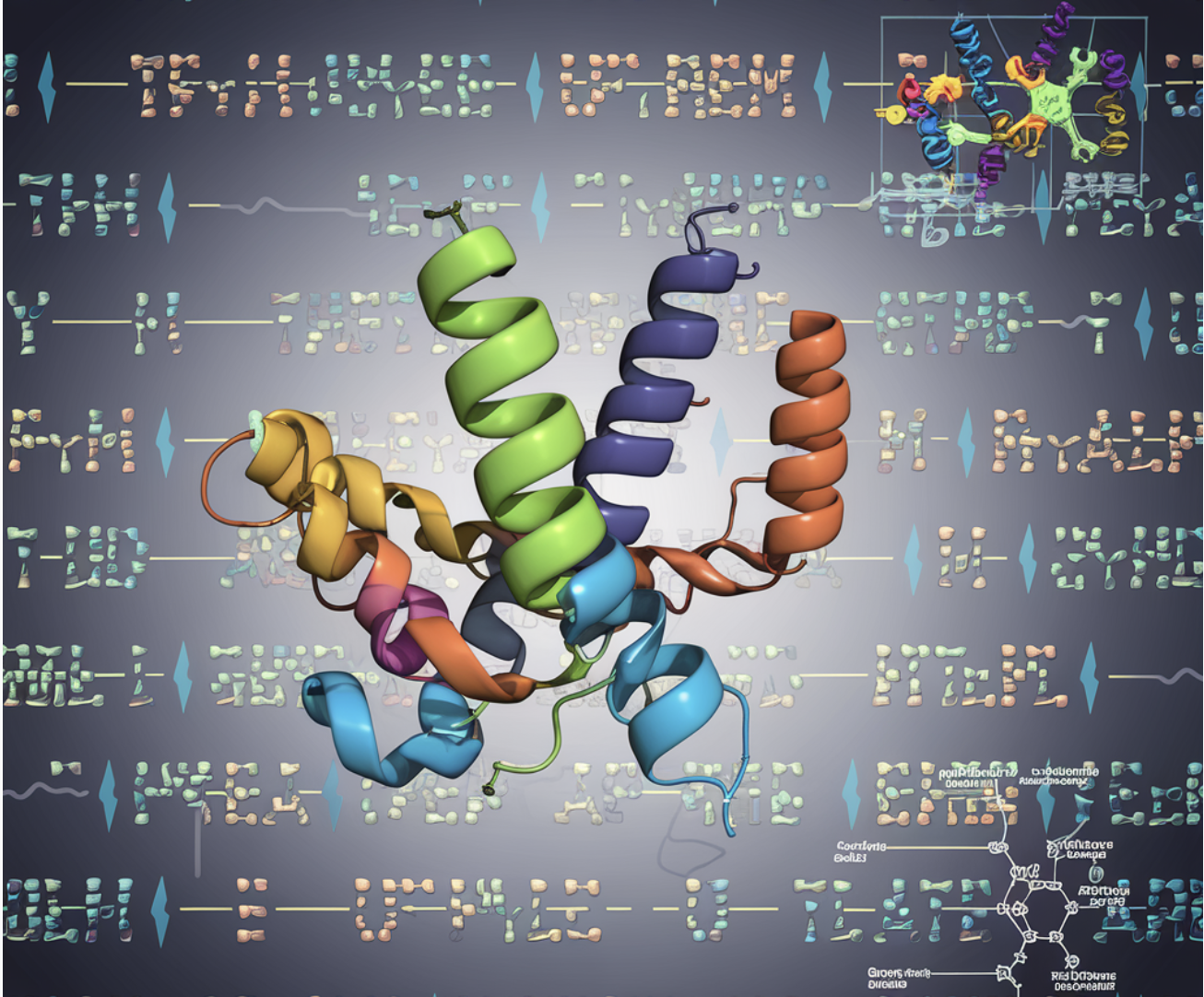
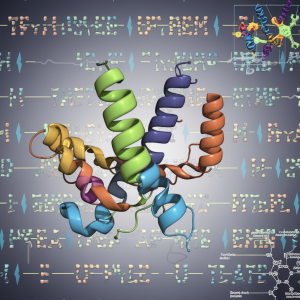
DeepMind has once again taken a significant step in computational biology with the release of AlphaFold 3’s inference codebase, model weights, and an on-demand server. This update brings unprecedented capabilities to the already transformative AlphaFold platform, extending its reach beyond proteins to accurately predict the structure and interactions of almost all of life’s molecules, including nucleic acids, ligands, ions, and modified residues, all in one unified platform. Let’s explore the implications and the technological leap represented by AlphaFold 3.
Addressing the Challenges in Biomolecular Structure Prediction
The accurate prediction of biomolecular structures is one of the most pressing challenges in biology and medicine. Complex biological processes, such as protein synthesis, signal transduction, and drug interactions, rely on intricate molecular structures and precise interactions. Despite significant advances with tools like AlphaFold 2, a considerable gap remained in modeling complexes that include various molecular types such as nucleic acids, ions, and other modifications. Traditional methods are typically domain-specific and fail to generalize well across diverse biomolecular entities. They also suffer from substantial computational requirements, resulting in delays that hinder quick experimentation and practical therapeutic design. To address these challenges, a more generalized, high-accuracy solution was needed—this is where AlphaFold 3 steps in.
DeepMind Releases AlphaFold 3
DeepMind recently released the inference codebase, model weights, and an on-demand server for AlphaFold 3. This release makes it easier for researchers and developers worldwide to integrate the power of AlphaFold into their workflows. Compared to its predecessor, AlphaFold 2, AlphaFold 3 offers a more sophisticated architecture capable of predicting the joint structure of biomolecular complexes, including proteins, DNA, RNA, ligands, ions, and even chemical modifications. This version is designed to accommodate highly complex interactions within biological systems, and the release includes access to model weights, allowing researchers to directly replicate or extend the existing capabilities.
The on-demand server makes AlphaFold 3 accessible without the need for substantial computational infrastructure. By simply providing sequence or structure input, users can query the server to obtain high-accuracy structural predictions, significantly lowering the barrier for research institutions and companies without advanced computational capabilities.
Technical Details
AlphaFold 3 introduces a diffusion-based architecture, significantly improving accuracy for predicting biomolecular interactions. Unlike AlphaFold 2, which mainly focused on proteins, AlphaFold 3 employs a generalized architecture capable of predicting structures for a broader range of biomolecular types. The new “pairformer” replaces AlphaFold 2’s “evoformer” as the central processing module, simplifying the process and improving efficiency. The system operates by directly predicting atomic coordinates using a diffusion model, removing the need for specific torsion angle predictions and stereochemical handling that added complexity in earlier models.
The multiscale nature of the diffusion process enhances the accuracy of predictions by reducing stereochemical losses and eliminating the need for multiple-sequence alignments. As shown in the benchmarks, AlphaFold 3 significantly outperforms traditional tools like AutoDock Vina and RoseTTAFold All-Atom, providing far greater accuracy in protein-ligand interactions and protein-nucleic acid complexes. These advancements not only make AlphaFold 3 more versatile but also drastically reduce the computational burden, allowing broader adoption across industries that need accurate biomolecular structures.
Importance of This Release
The release of AlphaFold 3 is monumental for many reasons. First and foremost, it fills a critical gap in our understanding of complex biomolecular interactions that involve not just proteins but multiple classes of molecules. The updated architecture of AlphaFold 3 can model almost any type of complex found in the Protein Data Bank (PDB). For instance, AlphaFold 3 demonstrated substantial improvement over previous versions, particularly in predicting antibody-antigen interactions, protein-ligand binding, and nucleic acid interactions with impressive accuracy across datasets like PoseBusters and CASP15 RNA targets. The performance metrics showed significant uplift across these tasks, with AlphaFold 3 achieving accuracy levels that outpaced traditional docking and nucleic acid prediction tools.
With improved on-demand availability, AlphaFold 3 empowers research into diseases that involve complex protein-DNA or protein-ligand interactions, such as cancer and neurodegenerative diseases, by providing reliable structural models for these intricate systems. Its ability to handle complex chemical modifications and predict accurate structures even in the presence of modifications (like glycosylation or phosphorylation) makes it invaluable for drug design and discovery. As such, AlphaFold 3 represents a step towards integrating computational models more effectively into therapeutic research, enhancing our capacity to design precise interventions at the molecular level.
Conclusion
DeepMind’s release of AlphaFold 3 has taken the world of structural biology into new territory. By including model weights, inference code, and an on-demand server, DeepMind has opened the door for researchers across disciplines to harness cutting-edge technology without prohibitive infrastructure requirements. AlphaFold 3’s advancements in structure prediction—spanning proteins, nucleic acids, ligands, and more—promise to accelerate our understanding of biomolecular interactions, potentially leading to significant breakthroughs in drug development and molecular biology.
Check out the Paper, Codebase, and Details. All credit for this research goes to the researchers of this project. Also, don’t forget to follow us on Twitter and join our Telegram Channel and LinkedIn Group. If you like our work, you will love our newsletter.. Don’t Forget to join our 55k+ ML SubReddit.
[AI Magazine/Report] Read Our Latest Report on ‘SMALL LANGUAGE MODELS‘
The post DeepMind Released AlphaFold 3 Inference Codebase, Model Weights and An On-Demand Server appeared first on MarkTechPost.