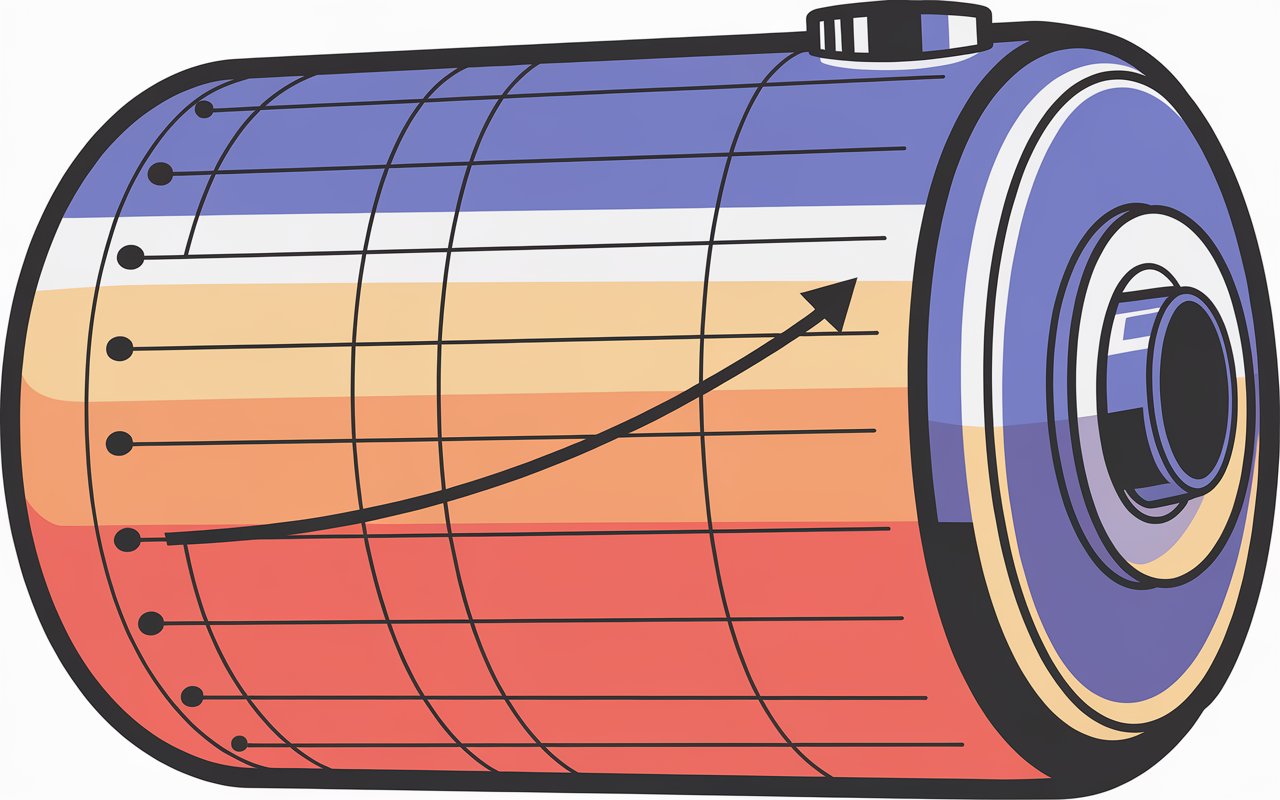
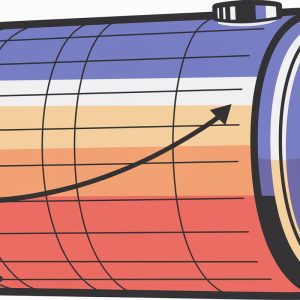
Predicting battery lifespan is difficult due to the nonlinear nature of capacity degradation and the uncertainty of operating conditions. As battery lifespan prediction is vital for the reliability and safety of systems like electric vehicles and energy storage, there is a growing need for advanced methods to provide precise estimations of both current cycle life (CCL) and remaining useful life (RUL).
Researchers from the Chinese Academy of Sciences, University of Waterloo, and Xi’an Jiaotong University addressed the critical challenge of accurately predicting the lifespan of lithium batteries, which is essential for ensuring the proper functioning of electrical equipment. Conventional approaches to battery lifespan prediction often rely on large datasets and complex algorithms, which are computationally intensive and lack flexibility across different operating conditions. These methods tend to struggle with generalization when applied to batteries using different charging strategies, making them less practical for real-world applications.
The researchers proposed a novel deep learning model, the Dual Stream-Vision Transformer with Efficient Self-Attention Mechanism (DS-ViT-ESA). This new model offers an innovative approach by using a vision transformer architecture combined with a dual-stream framework and efficient self-attention. The model was designed to predict both CCL and RUL of lithium batteries using minimal charging cycle data while maintaining high accuracy across various conditions, including unseen charging strategies.
The DS-ViT-ESA model leverages a vision transformer structure to capture complex, hidden features of battery degradation across multiple time scales. The dual-stream framework of the model processes the charging cycle data more effectively by separating the input into two streams. This allows a better understanding of the battery’s performance under different conditions. The efficient self-attention mechanism further enhances the model’s ability to focus on essential features within the data while minimizing computational cost.
The model requires only 15 charging cycle data points to achieve prediction errors of just 5.40% for RUL and 4.64% for CCL. Moreover, it demonstrated zero-shot generalization capabilities, which shows that it could accurately predict the lifespan of batteries subjected to charging strategies that were not part of the training dataset. This capability sets it apart from conventional methods, which often struggle with generalizing across different operating conditions. The model’s integration into the Battery Digital Brain system, called PBSRD Digit, has enhanced battery lifespan estimation’s overall accuracy and efficiency in large-scale commercial storage systems and electric vehicles.
In conclusion, the study provides a solution to the problem of accurately predicting lithium battery lifespan by presenting the DS-ViT-ESA model, which balances prediction accuracy and computational cost. The proposed method is innovative in using a vision transformer structure, dual-stream framework, and efficient self-attention mechanism, enabling highly accurate predictions with minimal data. By offering improved generalization and lower error rates, the model demonstrates significant potential for practical applications in energy management systems.
Check out the Paper. All credit for this research goes to the researchers of this project. Also, don’t forget to follow us on Twitter and join our Telegram Channel and LinkedIn Group. If you like our work, you will love our newsletter..
Don’t Forget to join our 50k+ ML SubReddit
The post Deep Learning Approach for Lithium-Ion Battery Life Prediction via Dual-Stream Vision Transformer appeared first on MarkTechPost.