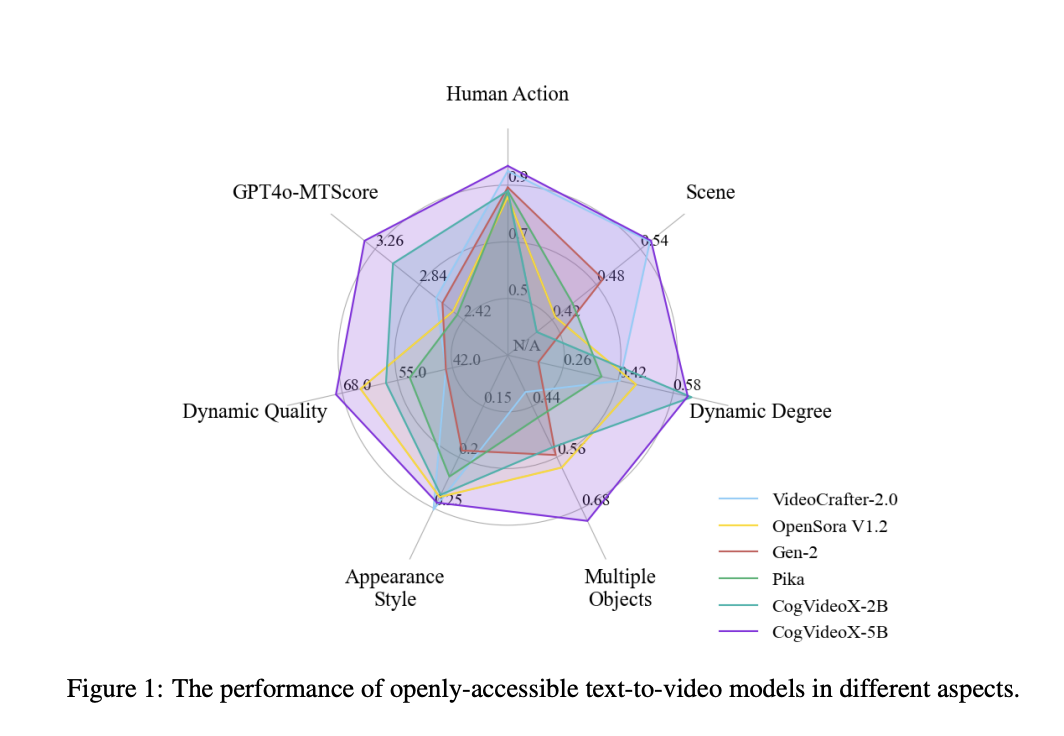
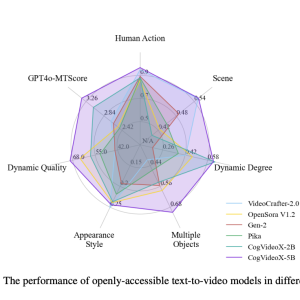
Text-to-video generation is rapidly advancing, driven by significant developments in transformer architectures and diffusion models. These technologies have unlocked the potential to transform text prompts into coherent, dynamic video content, creating new possibilities in multimedia generation. Accurately translating textual descriptions into visual sequences requires sophisticated algorithms to manage the intricate balance between text and video modalities. This area focuses on improving the semantic alignment between text and generated video, ensuring that the outputs are visually appealing and true to the input prompts.
A primary challenge in this field is achieving temporal consistency in long-duration videos. This involves creating video sequences that maintain coherence over extended periods, especially when depicting complex, large-scale motions. Video data inherently carries vast spatial and temporal information, making efficient modeling a significant hurdle. Another critical issue is ensuring that the generated videos accurately align with the textual prompts, a task that becomes increasingly difficult as the length and complexity of the video increase. Effective solutions to these challenges are essential for advancing the field and creating practical applications for text-to-video generation.
Historically, methods to address these challenges have used variational autoencoders (VAEs) for video compression and transformers for enhancing text-video alignment. While these methods have improved video generation quality, they often need to maintain temporal coherence over longer sequences and align video content with text descriptions when handling intricate motions or large datasets. The limitation of these models in generating high-quality, long-duration videos has driven the search for more advanced solutions.
Zhipu AI and Tsinghua University researchers have introduced CogVideoX, a novel approach that leverages cutting-edge techniques to enhance text-to-video generation. CogVideoX employs a 3D causal VAE, compressing video data along spatial and temporal dimensions, significantly reducing the computational load while maintaining video quality. The model also integrates an expert transformer with adaptive LayerNorm, which improves the alignment between text and video, facilitating a more seamless integration of these two modalities. This advanced architecture enables the generation of high-quality, semantically accurate videos that can extend over longer durations than previously possible.
CogVideoX incorporates several innovative techniques that set it apart from earlier models. The 3D causal VAE allows for a 4×8×8 compression from pixels to latents, a substantial reduction that preserves the continuity and quality of the video. The expert transformer uses a 3D full attention mechanism, comprehensively modeling video data to ensure that large-scale motions are accurately represented. The model includes a sophisticated video captioning pipeline, which generates new textual descriptions for video data, enhancing the semantic alignment of the videos with the input text. This pipeline includes video filtering to remove low-quality clips and a dense video captioning method that improves the model’s understanding of video content.
CogVideoX is available in two variants: CogVideoX-2B and CogVideoX-5B, each offering different capabilities. The 2B variant is designed for scenarios where computational resources are limited, offering a balanced approach to text-to-video generation with a smaller model size. On the other hand, the 5B variant represents the high-end offering, featuring a larger model that delivers superior performance in more complex scenarios. The 5B variant, in particular, excels in handling intricate video dynamics and generating videos with a higher level of detail, making it suitable for more demanding applications. Both variants are publicly accessible and represent significant advancements in the field.
The performance of CogVideoX has been rigorously evaluated, with results showing that it outperforms existing models across various metrics. In particular, it demonstrates superior performance in human action recognition, scene representation, and dynamic quality, scoring 95.2, 54.65, and 2.74, respectively, in these categories. The model’s ability to generate coherent and detailed videos from text prompts marks a significant advancement in the field. The radar chart comparison clearly illustrates CogVideoX’s dominance, particularly in its ability to handle complex dynamic scenes, where it outshines previous models.
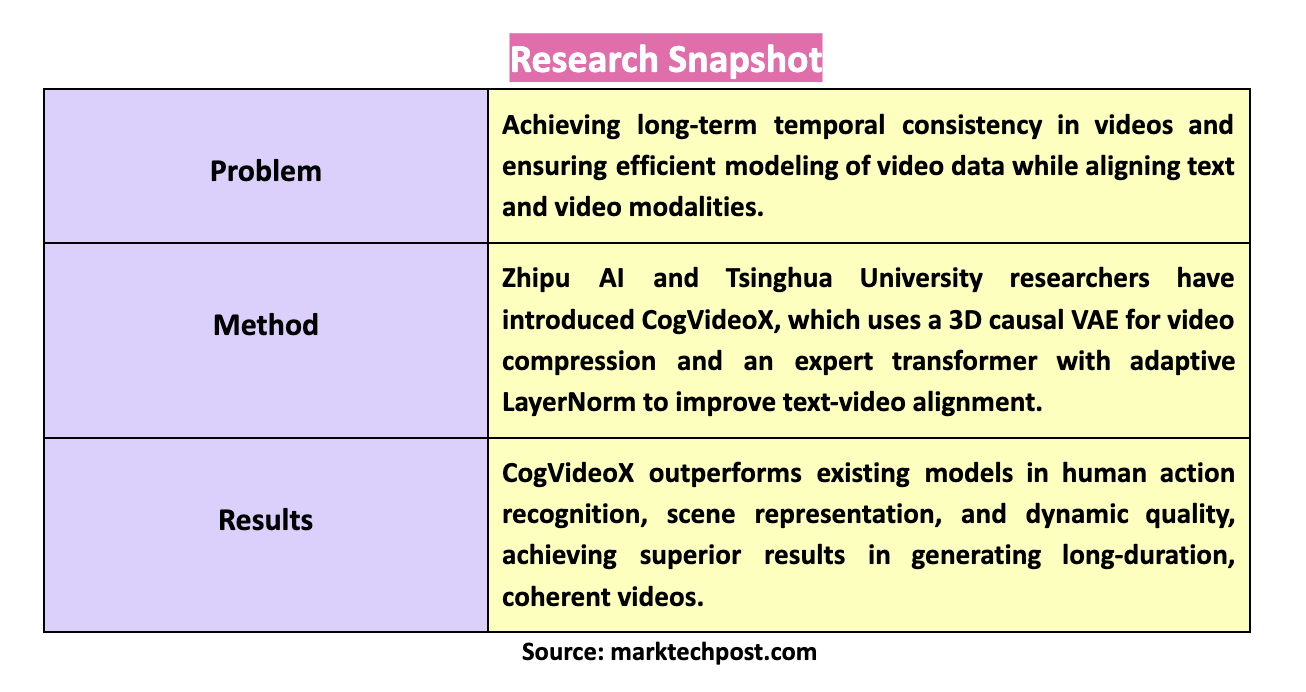
In conclusion, CogVideoX addresses the key challenges in text-to-video generation by introducing a robust framework that combines efficient video data modeling with enhanced text-video alignment. Using a 3D causal VAE and expert transformers, along with progressive training techniques like mixed-duration and resolution progressive training, allows CogVideoX to produce long-duration, semantically accurate videos with significant motion. Introducing two variants, CogVideoX-2B and CogVideoX-5B, offers flexibility for different use cases, ensuring that the model can be applied across various scenarios.
Check out the Paper, Model Card, GitHub, and Demo. All credit for this research goes to the researchers of this project. Also, don’t forget to follow us on Twitter and join our Telegram Channel and LinkedIn Group. If you like our work, you will love our newsletter..
Don’t Forget to join our 50k+ ML SubReddit
Here is a highly recommended webinar from our sponsor: ‘Building Performant AI Applications with NVIDIA NIMs and Haystack’
The post CogVideoX Released in Two Variants – CogVideoX-2B and CogVideoX-5B: A Revolutionary Advancement in Text-to-Video Generation with Enhanced Temporal Consistency and Superior Dynamic Scene Handling appeared first on MarkTechPost.