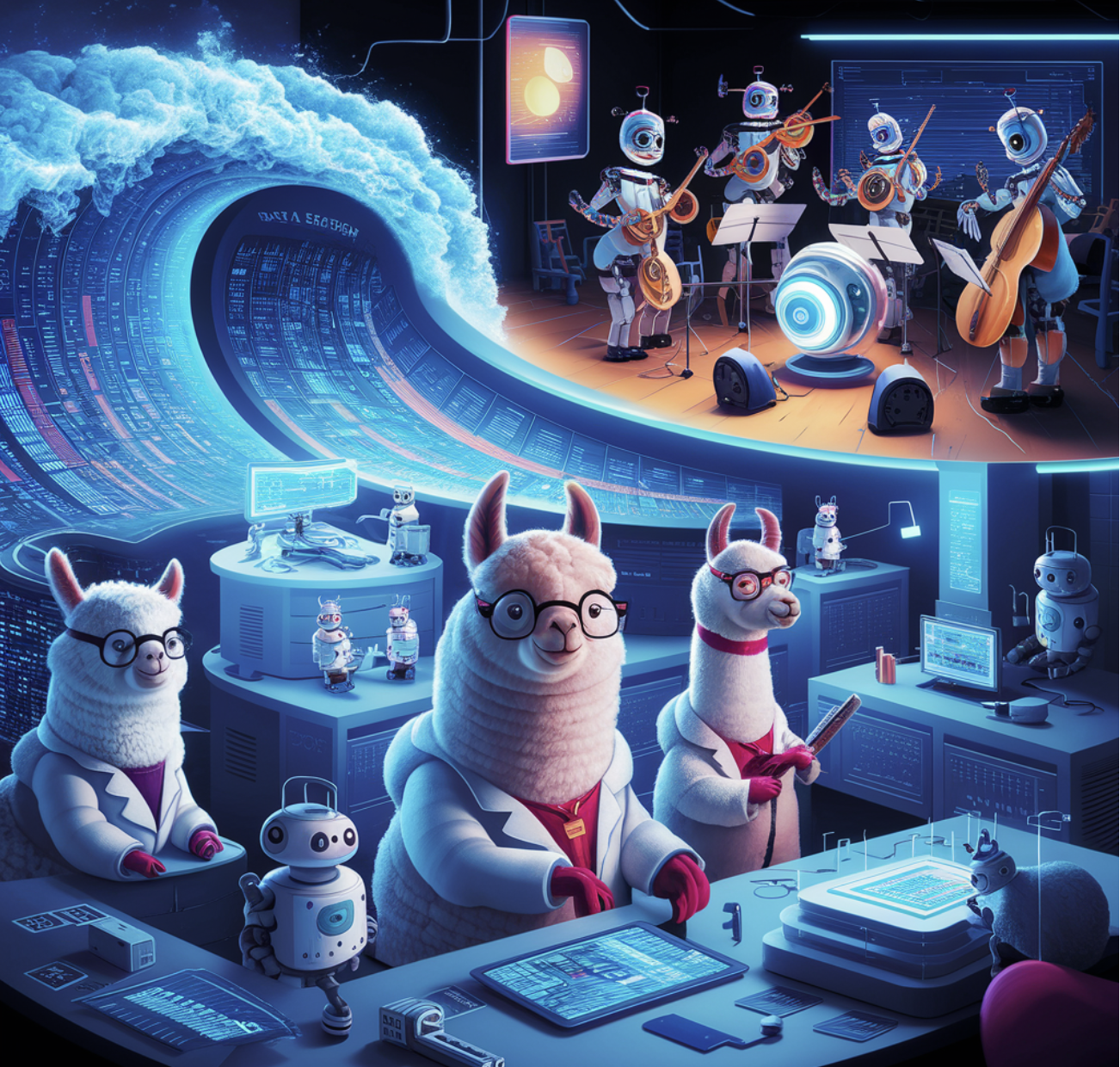
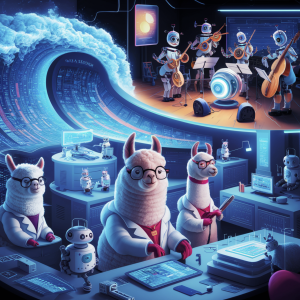
In the dynamic world of technology, Large Language Models (LLMs) have become pivotal across various industries. Their adeptness at natural language processing, content generation, and data analysis has paved the way for numerous applications. Let’s explore 15 detailed examples of how companies harness LLMs in real-world scenarios.
Netflix has shifted from traditional rule-based classifiers to machine learning-powered auto-remediation systems for handling failed big data jobs. This transition has enabled Netflix to automatically detect, diagnose, and fix issues in its data pipelines, significantly reducing downtime and ensuring seamless streaming services. The LLMs help understand log data, identify failure patterns, and suggest or implement fixes, thus enhancing operational efficiency and reliability.
Picnic, an online grocery delivery service, has integrated LLMs to improve the relevance of search results for product listings. Using large language models allows Picnic to better understand user queries and context, delivering more accurate and personalized search outcomes. This improvement enhances the customer experience and increases conversion rates by helping customers.
Uber’s advanced recommendation system personalizes out-of-app communications to enhance user engagement. Uber can tailor notifications and suggestions to individual user preferences and behaviors using sophisticated LLMs-powered recommender algorithms. This personalization extends beyond the app, ensuring that users receive relevant updates and offers via email, SMS, and other channels, thus improving user retention and satisfaction.
GitLab has developed GitLab Duo, a platform that validates and tests AI-generated outputs. This initiative uses LLMs to assess the quality, accuracy, and reliability of AI models at scale. GitLab Duo helps identify potential biases, errors, and areas for improvement in AI models, ensuring that the deployed models meet high performance and reliability standards. This rigorous testing process is crucial for maintaining trust in AI-driven features.
LinkedIn employs LLMs to recommend relevant premium products to its users. LinkedIn’s recommendation system can match members with the premium services and products that best suit their needs by analyzing user data, including professional history, interests, and activity patterns. This targeted approach helps LinkedIn enhance user satisfaction and drive subscriptions to its premium offerings.
Swiggy, a leading food delivery platform, utilizes hierarchical cross-domain learning to provide product recommendations to new users. By analyzing data from various domains and learning from user interactions, Swiggy’s recommendation system can offer personalized suggestions that cater to new users’ preferences. This approach effectively onboards new customers, increasing engagement and retention rates.
Careem, a ride-hailing service, leverages machine learning models to mitigate fraud risks through pre-authorization techniques. Careem can analyze transaction patterns and flag suspicious activities in real-time by implementing temporary holds on transactions. This proactive fraud detection mechanism, powered by LLMs, helps reduce fraudulent incidents, protecting the company and its users from potential losses.
Slack has developed AI capabilities that enhance secure and private corporate messaging. Utilizing LLMs, Slack’s AI features can process and analyze messages while ensuring high standards of security and privacy. These features include automated message summarization, smart replies, and context-aware suggestions designed to improve communication efficiency without compromising data protection.
Picnic has broken customer support language barriers using natural language processing (NLP). By routing support requests to the most suitable agents and providing real-time language translation, Picnic ensures that customers receive timely and accurate assistance regardless of language. This NLP-driven support system enhances customer service quality and helps Picnic cater to a diverse customer base.
Foodpanda employs machine learning to balance demand and supply for food delivery services. Using predictive analytics and advanced algorithms, Foodpanda can forecast demand patterns and allocate resources. This optimization helps manage delivery times, reduce operational costs, and ensure a better customer and delivery partner experience.
Etsy has implemented visual representation learning and evaluation techniques for visual search and similar recommendations. By leveraging computer vision and LLMs, Etsy’s system can analyze product images and provide users with visually identical items. This feature enhances the shopping experience by making finding products that match users’ preferences based on visual attributes easier.
LinkedIn has developed systems to detect AI-generated (deepfake) images. Utilizing advanced image recognition algorithms and LLMs, LinkedIn can identify and flag deepfake content, ensuring the integrity and trustworthiness of user-profiles and content on the platform. This capability is crucial in maintaining a secure and authentic user environment.
Discord, a popular communication platform, has explored various generative AI use cases to enhance user engagement. Discord can offer users creative tools such as AI-generated avatars, content moderation, and automated responses by rapidly developing and integrating generative AI features. These features leverage LLMs to improve user experience and foster a more interactive community.
Pinterest has evolved its ad conversion optimization models to enhance ad performance. By leveraging LLMs, Pinterest can analyze user behavior and preferences to deliver highly targeted & relevant ads. This optimization leads to higher conversion rates, better user experiences, and increased revenue for advertisers on the platform.
Expedia uses embeddings for lodging travel concepts to enhance its semantic search capabilities. By understanding the contextual meaning of user queries, Expedia’s search system can provide more accurate and relevant results for hotels and travel accommodations. This semantic search functionality, powered by LLMs, improves the booking experience by helping users find the best options based on their needs &preferences.
In conclusion, these examples illustrate the transformative impact of LLMs across various sectors, driving innovation and efficiency. As LLM technology advances, its applications are expected to expand, offering even more sophisticated solutions to industry challenges. Companies should consider leveraging dedicated platforms like AI Drive Pro to manage and optimize their LLM implementations for optimal results.
Note: This article is inspired from Evidently AI
The post 15 Real-World Examples of LLM Applications Across Different Industries appeared first on MarkTechPost.