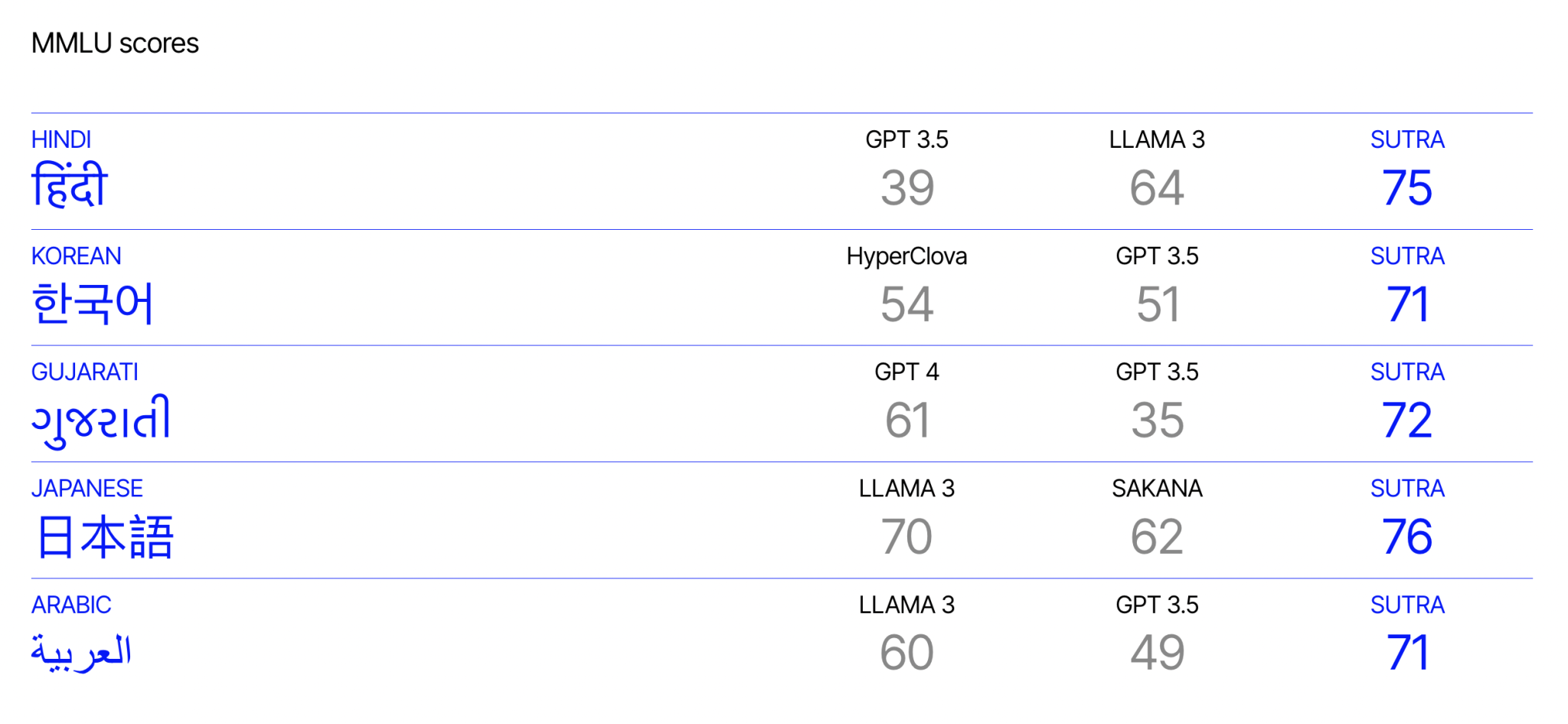
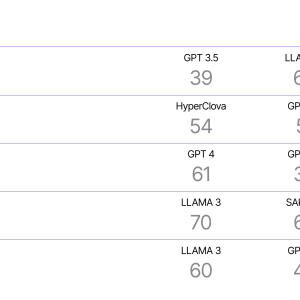
In the AI world, a new startup has emerged with the potential to reshape multilingual models, particularly in underserved regions. Two AI has launched SUTRA, a language model designed to be proficient in over 30 languages, including many South Asian languages such as Gujarati, Marathi, Tamil, and Telugu. This strategic move positions Two AI to address Southern Asia’s unique linguistic challenges and opportunities.
SUTRA’s architecture comprises two mixture-of-experts transformers: a concept model and an encoder-decoder for translation. The concept model is trained to predict the next token, leveraging publicly available datasets primarily in languages with abundant data like English. Concurrently, the translation model learned from 100 million human- and machine-translated conversations across multiple languages, allowing it to map concepts to similar embeddings in all languages it supports.
The innovative integration of these models involves the translation model’s encoder generating an initial embedding from the input text, which the concept model processes and feeds into the translation model’s decoder to produce the final output. This approach ensures that SUTRA can effectively handle a diverse range of languages, making it a robust tool for multilingual communication.
SUTRA is available in three versions: Pro, Light, and Online. SUTRA-Pro and SUTRA-Online offer high performance and internet connectivity at $1 per 1 million tokens, while SUTRA-Light provides a low-latency option at $0.75 per 1 million tokens. This pricing structure makes SUTRA an attractive option for users and businesses in cost-sensitive markets.
The model’s performance is particularly noteworthy. On the multilingual MMLU benchmark, which includes multiple-choice questions across various disciplines, SUTRA outperformed GPT-4 in four of the 11 reported languages: Gujarati, Marathi, Tamil, and Telugu. This demonstrates SUTRA’s strength in critical languages in the South Asian context. Furthermore, SUTRA’s tokenizer is highly efficient, producing fewer tokens than GPT-3.5 and GPT-4, especially in languages with non-Latin scripts like Hindi and Korean. This efficiency translates to faster and more cost-effective processing.
Despite its impressive capabilities, SUTRA’s evaluation of multilingual MMLU covers only 11 of its 33 languages, leaving its full multilingual potential somewhat uncharted. This limitation suggests that while SUTRA shows great promise, there is room for further validation and improvement across a broader range of languages.
Two AI’s strategic focus on non-English-speaking markets such as India, South Korea, Japan, and the Middle East highlights its ambition to cater to regions where English is not the predominant language. This focus is bolstered by significant seed funding of $20 million from Jio and Naver, indicating strong investor confidence in the company’s vision.
SUTRA, by offering a model that excels in local languages and is priced competitively, Two AI is well-positioned to carve out a niche in the AI market. SUTRA’s potential to provide high-quality, cost-effective multilingual support could bridge the gap for users in rural and underserved areas, bringing them closer to the benefits of cutting-edge AI technology.
In conclusion, while SUTRA may still need to match GPT-4 in all respects, its targeted performance, efficiency, and affordability make it a formidable competitor in the multilingual AI space. As Two AI continues to refine and expand SUTRA’s capabilities, it could play a pivotal role in the global AI landscape, particularly in regions historically overlooked by major AI developments.
Check out the Paper, Model, and Chatbot. All credit for this research goes to the researchers of this project. Also, don’t forget to follow us on Twitter.
Join our Telegram Channel and LinkedIn Group.
If you like our work, you will love our newsletter..
Don’t Forget to join our 45k+ ML SubReddit
The post Two AI Releases SUTRA: A Multilingual AI Model Improving Language Processing in Over 30 Languages for South Asian Markets appeared first on MarkTechPost.