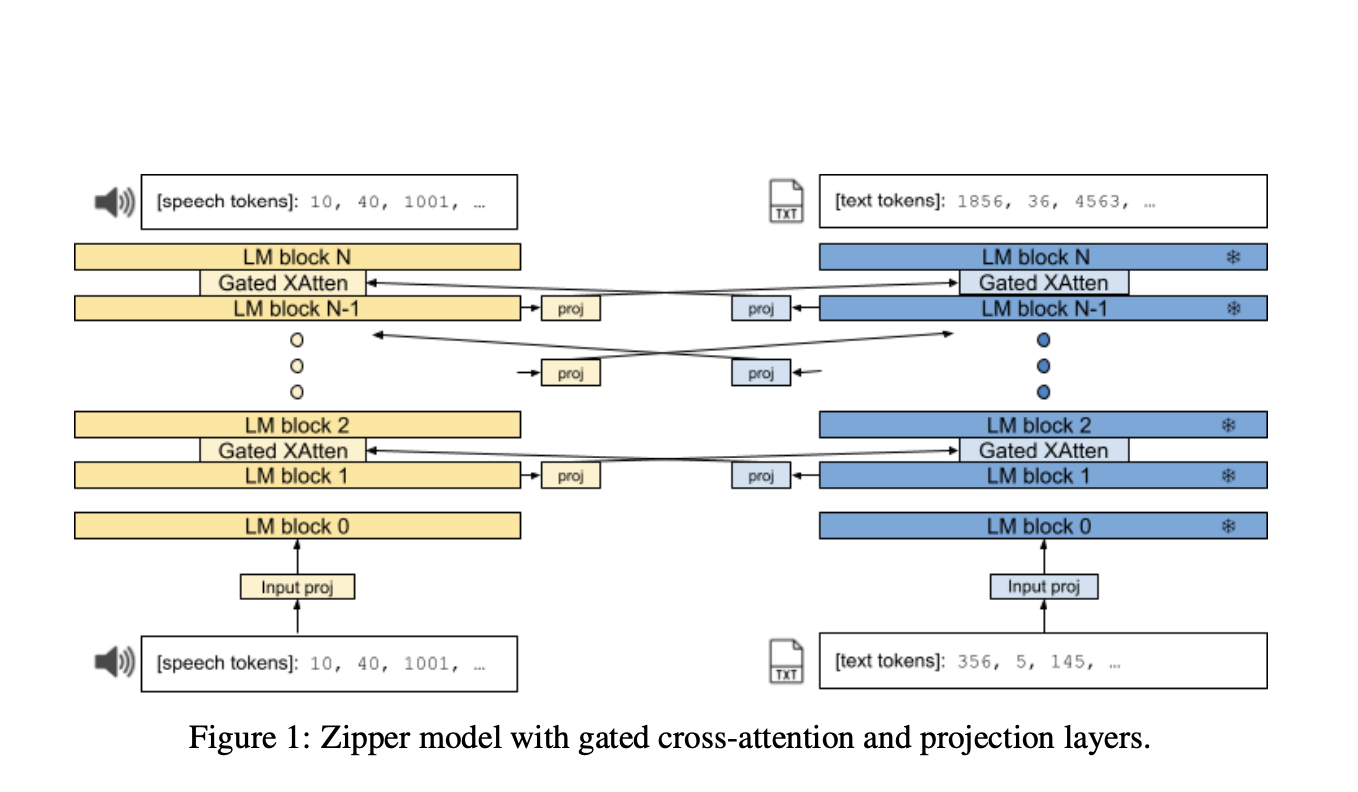
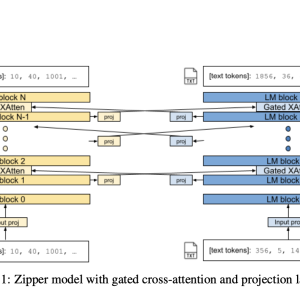
Integrating multiple generative foundation models helps by combining the strengths of models trained on different modalities, such as text, speech, and images, enabling the system to perform cross-modal tasks effectively. This integration allows for the efficient generation of outputs across multiple modalities simultaneously, leveraging the specific capabilities of each model. The two key issues in integrating multiple generative foundation models are the availability of aligned data across modalities and the effective utilization of unimodal representations in cross-domain generative tasks without compromising their original capabilities.
Google DeepMind researchers introduced Zipper to address the challenge of integrating multiple generative foundation models trained on different modalities into a unified framework beyond simple concatenation. Current approaches to multimodal generative models often rely on pre-training models with vocabulary expansion techniques or fine-tuning them on aligned multimodal data. However, these methods have drawbacks, including inflexibility in adding new modalities post-pre-training and the necessity for large quantities of aligned cross-modal data, especially when dealing with novel modalities. The proposed Zipper architecture, in contrast, offers a novel solution by leveraging independently pre-trained unimodal decoders and composing them using cross-attention mechanisms. This approach allows for the flexible reuse and re-purposing of pre-trained decoders while preserving unimodal performance.
The Zipper architecture consists of multiple autoregressive decoder towers, each independently pre-trained on a single modality using next-token prediction. These decoders are then combined using gated cross-attention layers, which enable the interchange of information between modalities at regular intervals. The architecture can equalize embedding dimension size differences and transform representations from one modality to another by inserting projection layers between modalities during cross-attention. During inference, the model generates output in the specified sequence of modalities until completion.
For the experiments to evaluate the proposed model, researchers used variants of PaLM2 models for the text backbone and a similar architecture for the speech backbone, pre-trained from scratch on the LibriLight dataset. Zipper’s competitive performance with the baseline indicates that freezing the text backbone does not significantly impact automatic speech recognition (ASR) performance. Zipper significantly outperforms the baseline for the Text-to-Speech, particularly when the speech backbone is unfrozen. These experiments highlight Zipper’s ability to preserve unimodal capabilities and better alignment capabilities of cross-attention. Zipper was able to achieve meaningful results with just 1% of the original training data, demonstrating superior performance with significantly less aligned data,
In conclusion, the Zipper architecture offers a flexible and scalable solution for integrating independently pre-trained unimodal decoders. Zipper uses cross-attention mechanisms to make modality composition work well even without extensive aligned data. It also keeps unimodal performance high while getting competitive results in cross-modal tasks. This approach could advance multimodal generative modeling across various domains and pave the way for future research combining more modalities.
Check out the Paper. All credit for this research goes to the researchers of this project. Also, don’t forget to follow us on Twitter. Join our Telegram Channel, Discord Channel, and LinkedIn Group.
If you like our work, you will love our newsletter..
Don’t Forget to join our 43k+ ML SubReddit | Also, check out our AI Events Platform
The post Google DeepMind Introduces Zipper: A Multi-Tower Decoder Architecture for Fusing Modalities appeared first on MarkTechPost.